Exploring AI Art Styles: A Guide
In the burgeoning sphere of artificial intelligence, the emergence of neural network AI art styles represents a confluence of technology and creativity, reshaping the landscape of visual expression. This guide endeavors to demystify the complexities behind the algorithms that enable machines to emulate and innovate upon human artistry.
With a focus on pioneering methods such as DeepDream’s hallucinogenic visuals and StyleGAN’s lifelike textures, the discussion extends to the broader implications these technologies have across industries, from entertainment to design.
As we examine the balance between content and style loss, the intricacies of generative adversarial networks (GANs), and their creative diversity, one must ponder the ethical considerations and legal ramifications accompanying this digital renaissance.
The following discourse will elucidate the mechanisms powering AI-generated art and contemplate its place in the continuum of artistic evolution.
Key Takeaways
- Neural networks, such as Convolutional Neural Networks (CNNs) and Neural Style Transfer (NST), are critical techniques for creating AI art.
- Neural Style Transfer (NST) allows for integrating style elements from one image with the content of another, enabling creators to blend and transform images in innovative ways.
- DeepDream generates psychedelic visuals by amplifying and extrapolating patterns in an image, creating a unique and otherworldly aesthetic.
- StyleGAN utilizes layered neural network architecture and advanced gram matrices to generate textures with remarkable detail and lifelike accuracy, enhancing creations with a level of realism previously unattainable.
Understanding Neural Networks
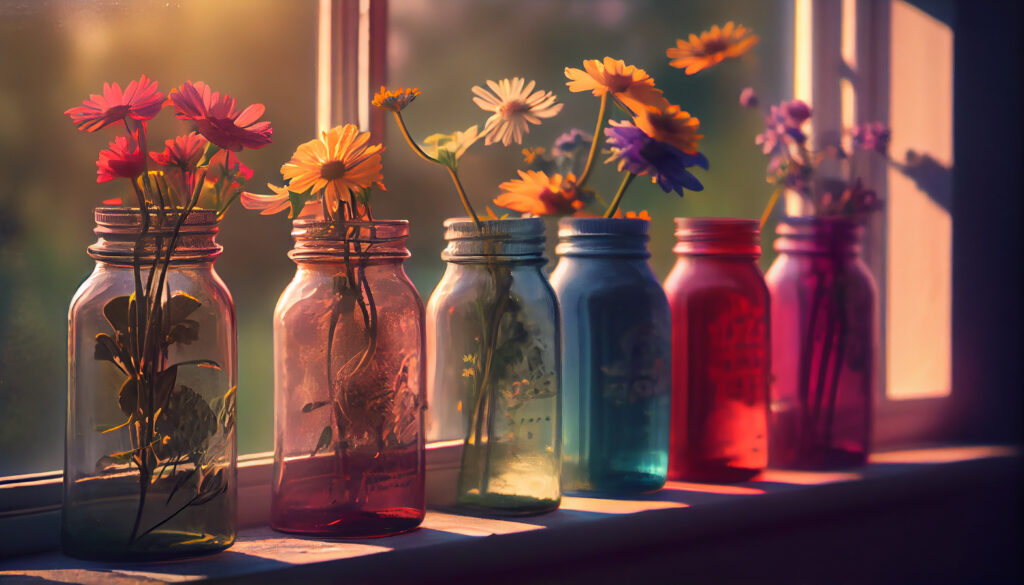
To fully appreciate the intricacies of Neural Style Transfer (NST), one must first comprehend the fundamental workings of neural networks, which are complex computational systems inspired by the human brain’s network of neurons. These networks, pivotal in Artificial Intelligence (AI), form the backbone of NST. Through Deep Learning, neural networks can learn and identify intricate patterns in data, enabling advanced applications in computer vision and beyond.
Central to NST are Convolutional Neural Networks (CNNs), a class of Deep Neural Networks specifically designed to process pixel data. These networks employ layers of artificial neurons to perform convolutions across an image, extracting and learning a hierarchy of feature representations. The effectiveness of NST is contingent upon these known representations, which encapsulate the essence of the content and style of input images.
During the NST process, a pre-trained model, typically a Deep Convolutional network, is utilized to evaluate content and style loss functions. These functions measure the divergence between the generated and the source images, guiding the transfer mechanism. The content loss ensures fidelity to the original image’s structure, while the style loss quantifies adherence to the aesthetic patterns of the style image. Incorporating Total Variation loss further refines output by diminishing noise, thus enhancing the visual coherency of the synthesized artwork.
Pioneering AI Art Methods
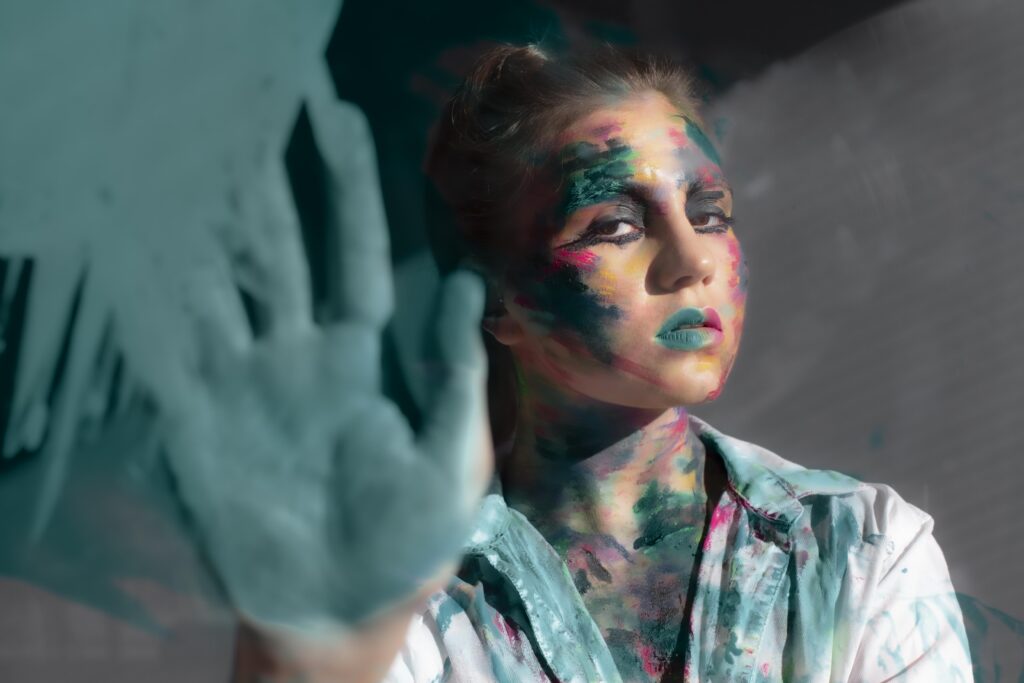
Building upon the foundation of neural network capabilities, AI art methods like Neural Style Transfer have emerged, revolutionizing how we create and interact with digital art. Neural Style Transfer (NST) is a technique that integrates the stylistic elements of one image (the style image) with the content of another (the content image), effectively redefining the aesthetic representation of the generated image. This process leverages the power of Convolutional Neural Networks, particularly employing the VGG network architecture pre-trained on the ImageNet dataset.
The technical intricacies of style transfer models are grounded in the computation of Content Loss and Style Loss. Content Loss ensures the generated image retains the essence of the original content image, while Style Loss, calculated using a gram matrix, aims to match the artistic styles reflected in the style image.
Critical considerations in NST include:
- Arbitrary style transfer: The ability to apply artistic style to a content image without retraining the model.
- Optimization of style loss: Techniques to minimize the difference between the generated and style images‘ styles.
- Preservation of content: Balancing the content and style to maintain the recognizability of the original content image.
Through these methods, NST has facilitated a new form of artistic expression, enabling creators to blend and transform images in once unthinkable ways.
DeepDream’s Psychedelic Imagery
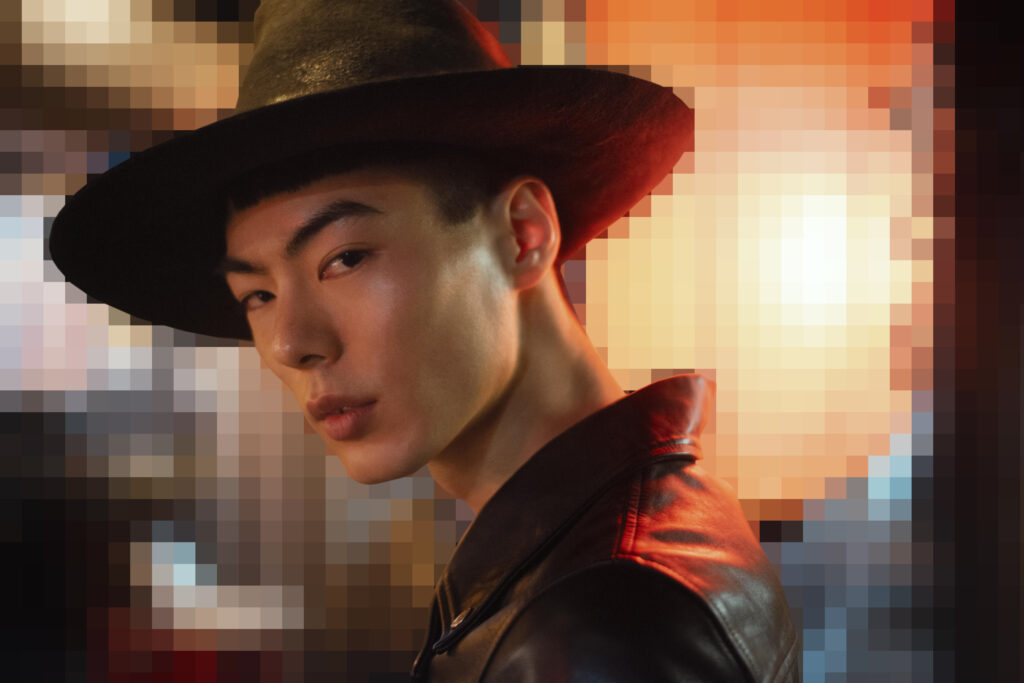
DeepDream, an art style generated by neural networks, is renowned for its distinctive psychedelic visuals that transform mundane images into extraordinary, dream-like creations. This technique leverages the inherent capacity of neural networks to amplify and extrapolate patterns and features within an image, producing surreal and intensely intricate effects.
Generating DeepDream’s psychedelic imagery involves submitting a base image to a neural network, which then selectively enhances specific patterns—often those that resemble natural or biological forms. The result is a style image that bursts with vibrant colors and complex, recursive patterns.
The artistic application of DeepDream extends beyond simple pattern recognition; it also encompasses style transfer, where the style information from one image is applied to another. However, DeepDream’s approach is unique in not relying on a separate style image. Instead, it iterates upon the content of the original image to generate the distinctive features of its hallucinatory aesthetic.
DeepDream’s psychedelic imagery has captivated audiences with its ability to render the familiar as otherworldly, encouraging viewers to question the boundaries between the artificial and the organic in visual art.
StyleGAN’s Realistic Textures
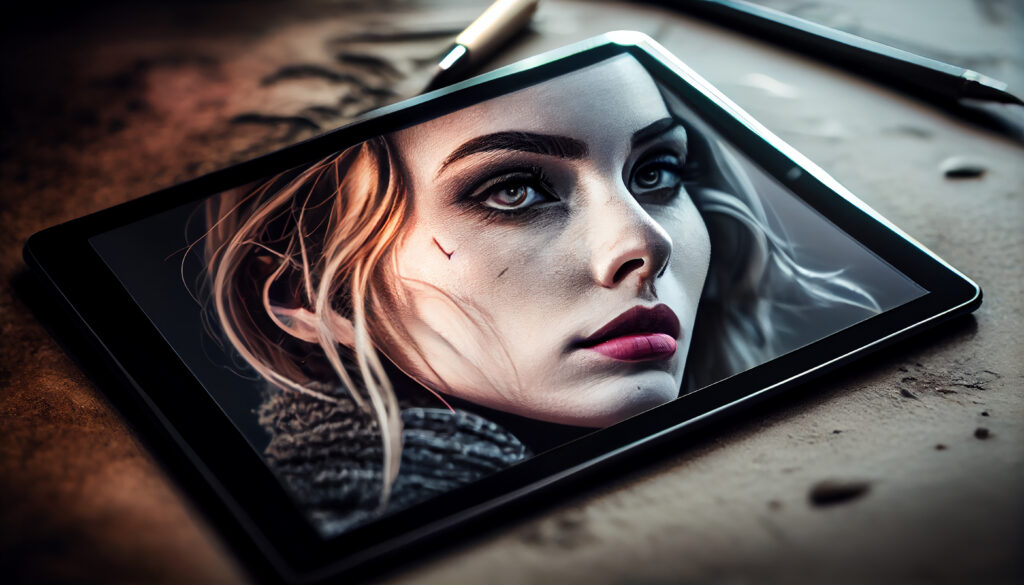
While DeepDream’s psychedelic imagery is captivating with its dream-like distortions, StyleGAN’s realistic textures represent another striking capability of neural networks, showcasing an ability to generate textures with remarkable detail and lifelike accuracy. Leveraging the sophistication of Generative Adversarial Networks, StyleGAN’s realistic textures are at the forefront of AI art generators. These synthesizing textures can fool the human eye into believing they are genuine materials.
The technical prowess behind StyleGAN’s realistic textures stems from:
- Layered Neural Network Architecture: The deep structure of StyleGAN allows for a granular approach to style transfer, where content and style can be intricately balanced.
- Advanced Gram Matrices: Using gram matrices, StyleGAN captures style features from a specific image and applies them to the target content, retaining the original structure while infusing new textural details.
- Pre-trained VGG Networks: Using pre-trained VGG networks enables StyleGAN to generate high-quality textures by drawing on a vast repository of learned style data.
This AI-driven method creates authentic textures, from the softness of animal fur to the complex weaves of fabric and the nuanced variations in natural elements.
Designers and artists can employ StyleGAN to impart depth and authenticity, enhancing their creations with a level of realism previously unattainable through traditional digital means.
GANs and Creative Diversity
Generative Adversarial Networks (GANs) have revolutionized the field of AI art by enabling unprecedented creative diversity through their complex interplay of generative and discriminative components.
At the heart of this innovation are two neural networks: the generator, which creates art, and the discriminator, which evaluates it. The generator crafts images by learning the intricate parameters of the style from a vast dataset, while the discriminator ensures the quality by challenging the generator to produce high-quality AI art that is indistinguishable from human-made work.
The capacity of GANs to perform style transfer—where the style image’s characteristics are imbued into the generated art—exemplifies their ability to capture style nuances and image features with remarkable precision. As the generator iterates through the training data, it uncovers and assimilates diverse artistic styles, resulting in the ability to generate an image that is both novel and a tribute to the style image.
This dynamic process leads to endless possibilities in AI-generated art, with GANs constantly pushing the boundaries of what can be achieved. The diversity is in mimicking existing styles and creating new ones, thus expanding the creative horizons of artists and technologists alike.
FAQs
What does the term “Neural Network AI Art Styles” refer to?
Neural Network AI Art styles” implies a guide that explores various artistic styles created or influenced by neural networks, a type of artificial intelligence. The guide likely covers different techniques, models, and approaches for generating diverse art styles.
How do neural networks contribute to the creation of different art styles?
Neural networks contribute to creating different art styles by learning patterns from diverse datasets and generating new images or artworks that reflect the characteristics of those styles. This can include anything from classical art styles to more contemporary or abstract forms.
Are there specific types of neural networks commonly used for generating art styles?
Certain types of neural networks, such as Generative Adversarial Networks (GANs) and Variational Autoencoders (VAEs), are commonly used for generating art styles. GANs, in particular, have been widely employed for their ability to produce visually appealing and diverse results.
Can artists control or customize the output of neural networks for specific art styles?
Yes, artists can often control or customize the output of neural networks for specific art styles by adjusting various parameters input data, or by using conditional models that allow for more targeted style generation.
How diverse are the art styles that can be generated using neural networks?
The diversity of art styles that can be generated using neural networks is vast. Neural networks can replicate traditional art styles, mimic famous artists, or even create entirely new and abstract styles, showcasing the flexibility and adaptability of these models.