Mastering AI Art: 100% Neural Techniques
In the rapidly evolving landscape of artificial intelligence, the intersection of AI and art has given rise to a new paradigm of creative expression. The course, ‘100% Neural: Mastering AI Art Generator Techniques’, explores the tools and methodologies that empower artists and technologists to forge this avant-garde genre.
As we embark on a journey through the intricacies of generative adversarial networks (GANs), diffusion models, and neural style transfers, participants will be equipped with the knowledge to craft visually stunning pieces that challenge the traditional boundaries of art.
The ethical implications of AI-generated art are as complex as the algorithms themselves, raising questions that demand our attention. By examining these considerations alongside enhancing creativity and innovation, this course offers a comprehensive look into the mastery of AI art generation. This field is reshaping aesthetics and the artist’s role in the age of algorithms.
To fully grasp the magnitude of this shift, one must consider the broader impacts and the potential that lies within the synthesis of neural networks and human creativity.
Key Takeaways
- AI art generation relies on complex algorithms and neural networks.
- Generative AI facilitates the emergence of novel artwork.
- GANs and diffusion models are essential techniques in AI art generation.
- Ethical considerations surrounding AI art include copyright issues, authenticity, deception, societal impact, and transparency.
Understanding AI Art Basics
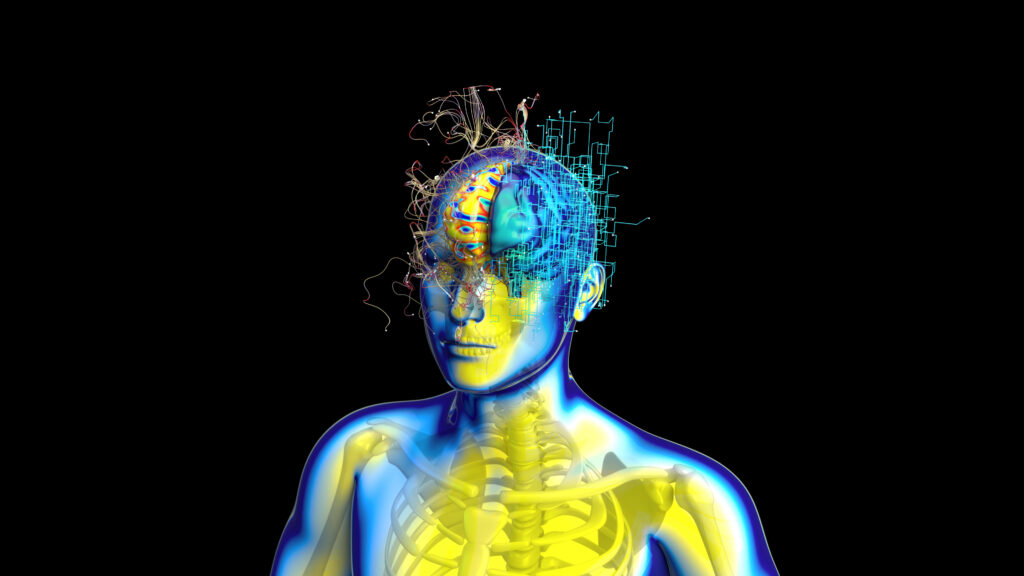
At the intersection of machine learning and visual creativity, AI art generation fundamentally relies on complex algorithms and neural networks to transform textual or data inputs into unique visual representations. This multidisciplinary domain harnesses artificial intelligence (AI), particularly Generative AI, to facilitate the emergence of novel artwork through techniques like style transfer and the interpretation of text prompts. Deep Learning courses often elucidate the intricacies of these processes, emphasizing the roles of artificial neural networks in image generation.
Technologies pivotal to AI Art Generation employ models such as Contrastive Language-Image Pre-training (CLIP) and Natural Language Processing (NLP) to interpret and visualize concepts. Generative Adversarial Networks (GANs) are a cornerstone of this technological framework, wherein a generator network crafts images from latent space, which undergoes scrutiny by a discriminator network that distinguishes between authentic and generated samples. This dynamic competition drives the refinement of synthetic images to a high fidelity.
Diffusion models represent an advanced generator class synthesizing contextually relevant and aesthetically compelling images. These models progressively build upon random noise to achieve a coherent output guided by the probabilistic understanding of the data distribution. Mastery of AI Art Generation thus necessitates a deep technical comprehension of these techniques and their underlying principles.
Tools and Software Overviews
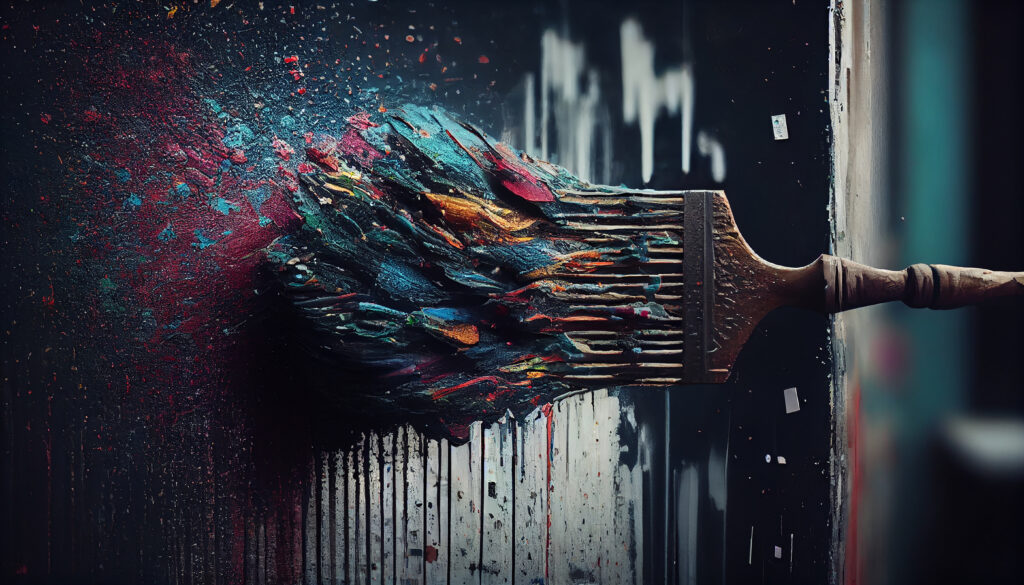
Delving into the realm of AI art generation, many tools and software stand ready to transform creative visions into digital reality through advanced neural networks and generative models. Stable Diffusion and Generative Adversarial Networks (GANs) are at the forefront, leveraging image processing techniques to create intricate visuals. These software applications harness the power of computer vision and deep learning to generate increasingly indistinguishable images from those made by human artists.
Artists and developers can use AI-generated art tools to explore the open-source nature of many platforms, thereby democratizing the field. For example, diffusion models have become famous for their ability to produce high-quality images from natural language descriptions, intertwining with GPT language models to enhance the generation process. This synergy enables the production of aesthetically pleasing and semantically coherent visuals.
Exploring these tools reveals the underlying neural networks, such as LSTMs, which contribute to the temporal coherence in sequential art generation. Aspiring generative artists can access extensive resources, including over 50 Google Colab notebooks illustrating various algorithms and techniques. Through these technical avenues, one can gain insights into the intricacies of AI art generation and refine their mastery of the digital canvas.
Crafting Techniques With GANs
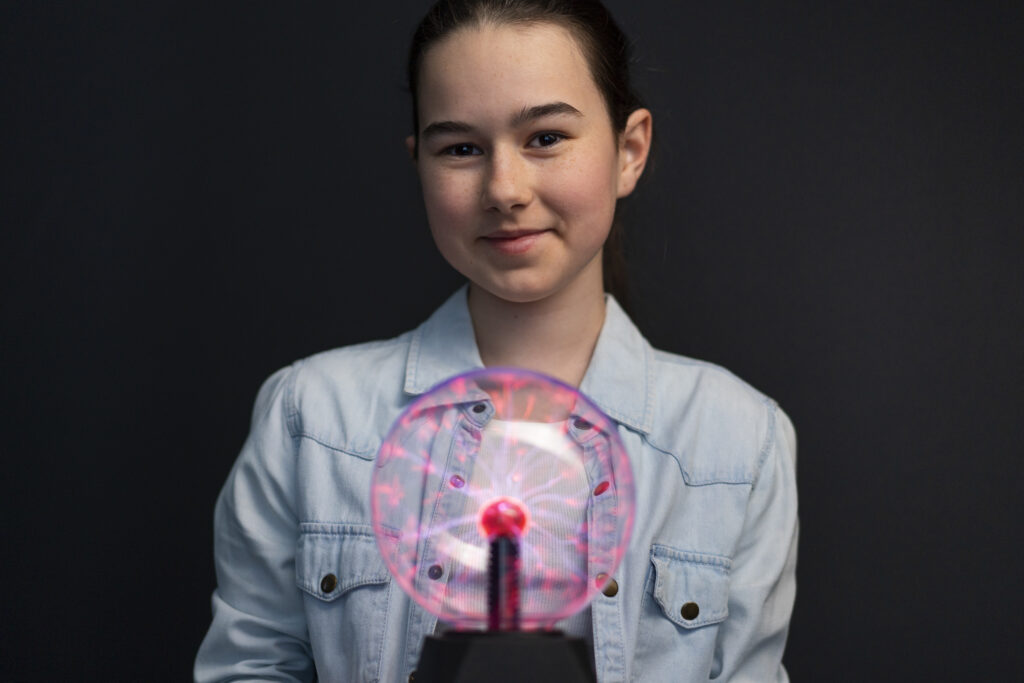
Generative Adversarial Networks (GANs) employ a dual-network architecture to refine synthetic outputs toward realism iteratively in AI art generation.
Exploring the foundational structure of GANs elucidates the dynamics between generators and discriminators, which is critical for optimizing the fidelity of generated images.
Advances in enhancing GAN outputs focus on fine-tuning these interactions, thereby improving the quality and diversity of artificial artwork.
GAN Basics Explained
Generative Adversarial Networks (GANs) fundamentally alter the landscape of machine learning by pitting two neural networks against each other in a strategic game that enhances their ability to generate remarkably realistic synthetic data. By leveraging architectures rooted in computer vision and deep learning, GANs employ a generative model to create images using complex A.I. techniques.
The generator crafts data from random noise aiming to fool the discriminator—a binary classifier tasked with discerning the authenticity of the samples. This adversarial process iteratively refines the output, yielding advanced techniques for generating images that often rival authentic data in verisimilitude.
Contrasting GANs, diffusion models represent an alternative approach, progressively transforming noise into structured, contextually relevant art. These refined A.I. methodologies exemplify the profound capabilities of GANs in the domain of A.I.-generated art.
Enhancing GAN Outputs
To enhance the outputs of GANs, artists, and developers often refine the training process and algorithm parameters to achieve higher-quality, more intricate art generation. By manipulating these elements, they ensure the generation of authentic images that reflect the nuanced aesthetics found in the real world.
The Midjourney of mastering these techniques involves a dedicated practice that can be facilitated through structured learning.
- Utilize advanced training techniques to Create Visually Stunning outcomes
- Fine-tune GAN parameters for generating authentic images
- Offer rich content with real-world examples in educational courses
- Continuously learn and adapt to new GAN advancements
- Invest in the Image Course to master creating unique, high-quality images
This analytical approach empowers creators to produce captivating visuals that resonate emotionally with viewers.
Advancing With Diffusion Models
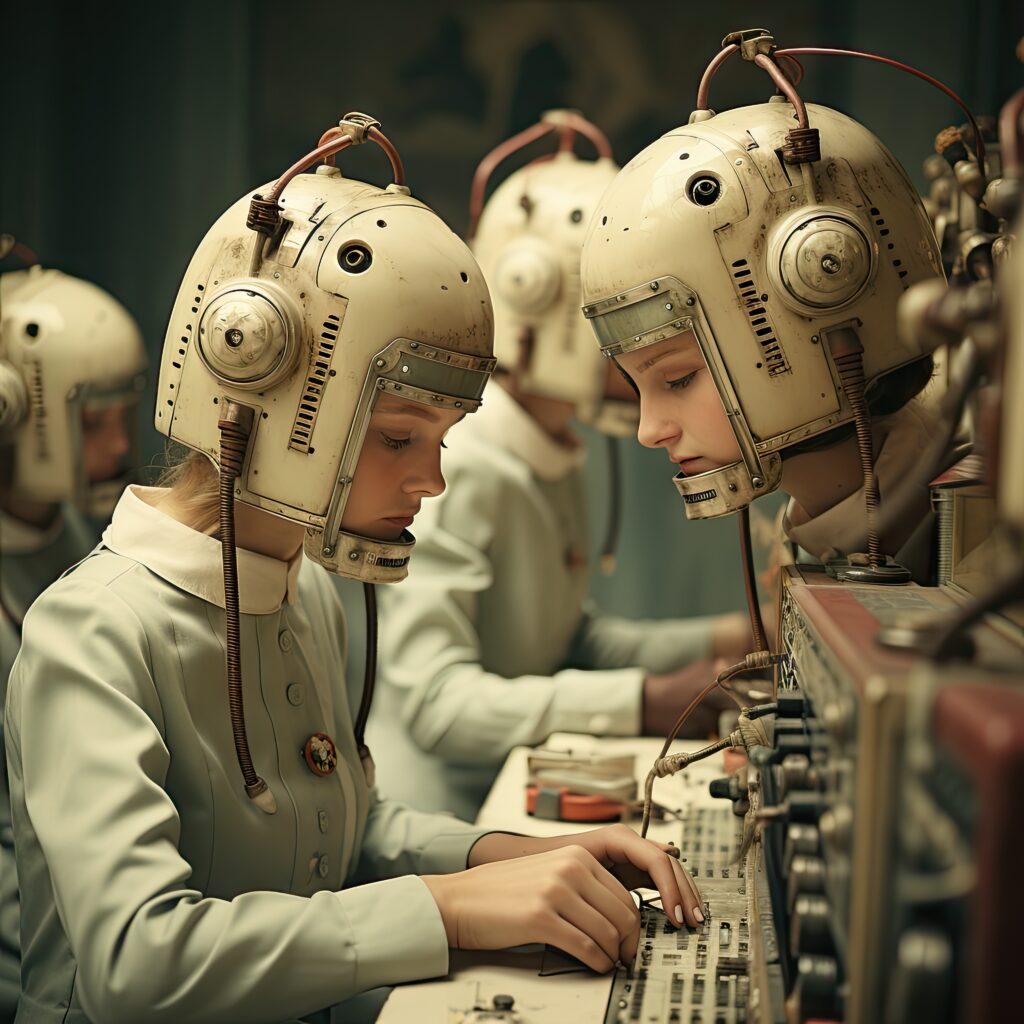
Diffusion models represent a groundbreaking shift in AI art generation, utilizing particle simulation techniques to craft aesthetically compelling and contextually coherent images. At the intersection of computer science and machine learning, these models epitomize the cutting edge Reinforcement of generative algorithms. They transform noise into structured images by progressively refining random data points, mirroring the physical diffusion process.
These models synergize with the Contrastive Language-Image Pre-training (CLIP) language model, enhancing text understanding to ensure relevance in the AI-generated images. Diffusion models can interpret nuanced prompts through this fusion, translating abstract concepts into detailed visual representations.
Learning how to train these models is now increasingly accessible through comprehensive course bundles, which provide structured learning paths for enthusiasts and professionals alike.
Using advanced infrastructure, often cloud-based, has democratized the creation process, allowing artists and developers to generate new images without prohibitive hardware investments. This paradigm shift has had significant implications in creative industries, where diffusion models are leveraged to visualize novel product designs swiftly and conjure realistic environments and characters for interactive media such as video games and movies.
Neural Style Transfer Applications
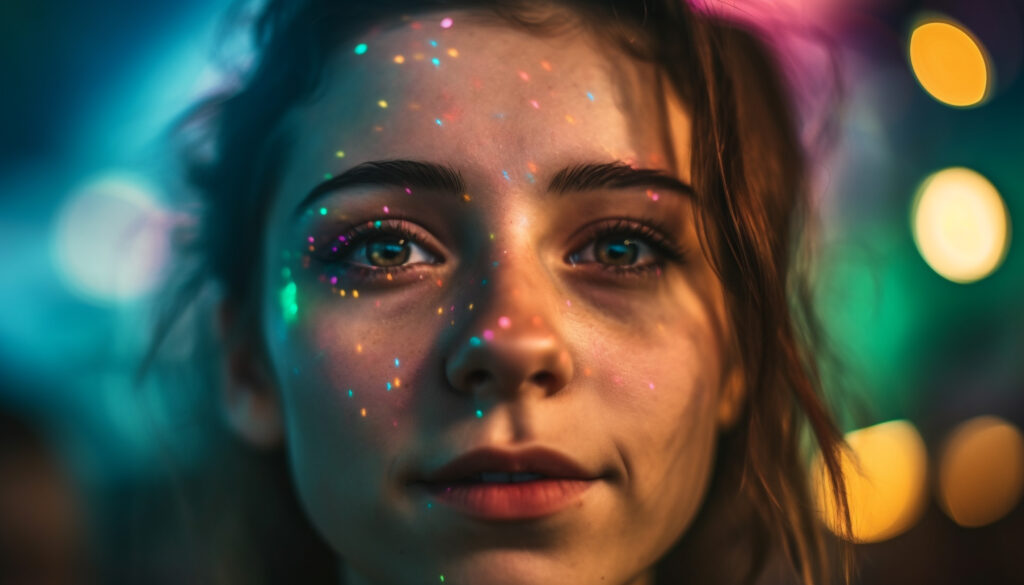
Neural style transfer applications have revolutionized the field of creative imagery by allowing the seamless fusion of artistic styles and thematic elements within a wide array of visual content. These methods have advanced to a point where they create visually stunning pieces and contribute significantly to various sectors that rely on visual creativity.
- It accelerated product design by rapidly generating multiple visual iterations.
- It enhanced video game and movie environments with stylistically adapted elements that resonate with audiences.
- Innovative marketing visuals that capture consumer attention with a unique aesthetic appeal.
- Artistic expression democratized, enabling creators to produce artwork that embodies deep cultural and historical art styles.
- Educational tools that make learning about art history interactive and engaging through visual examples.
These applications exemplify the mix of rich content and technical sophistication that is well organized and conceived. They are helpful without just handing solutions, encouraging users to engage deeply with the content. Using multiple neural networks, these tools provide cloud-based execution that ensures fast response to questions and assignment grading. The depth of information supplied is balanced—light enough to understand and challenging enough to inspire further exploration.
Ethical Considerations of AI Art
As AI-generated art becomes increasingly prevalent, it raises complex ethical issues concerning copyright, authenticity, and the ramifications of its use in society. Deploying artificial intelligence in the creative realm necessitates rigorous scrutiny to uphold integrity and respect for intellectual property. This table outlines critical ethical considerations:
Ethical Concern | Description | Potential Solutions |
---|---|---|
Copyright | Ownership of AI-generated art is contentious. | Implement digital certificates to establish provenance. |
Authenticity | Distinction between human-made and AI art is blurred. | Ensure clarity of explanations provided for AI contributions. |
Deception | AI art could be used for fraudulent purposes. | Monitor usage and apply regulations to prevent misuse. |
Societal Impact | AI may perpetuate biases in its creations. | Review and correct algorithmic biases; promote diversity. |
Transparency | Unclear process of AI art generation. | Provide detailed content for each lesson on the AI’s workings. |
In developing a language learning course on AI art generation techniques, mentioning the clarity of the video lectures and the relevance of good real-world examples is pivotal. The “Next amazing thing” in AI art must be delivered with an analytical mindset, ensuring each lesson segment contributes constructively to the overarching narrative. By doing so, the course is well-positioned to educate responsibly, nurturing informed, ethical AI art practitioners.
Enhancing Creativity and Innovation
AI art generators catalyze innovation by enabling artists to transcend traditional constraints and explore uncharted aesthetic territories.
These tools can amplify creativity by integrating diverse perspectives and leveraging collaborative efforts, yielding novel and complex art forms.
A continuous learning paradigm, underpinned by these AI systems, challenges established norms and facilitates the emergence of groundbreaking artistic expressions.
Fostering Artistic Exploration
Exploring the fusion of styles, concepts, and attributes through AI image generators opens new avenues for artistic creativity and innovation. As artists and educators delve into these tools, they can create unique pieces that showcase their perspective and enhance their understanding of AI’s evolving capabilities. By engaging with AI art generators, users can learn the content of Language Learning in a visual context, build on the concepts with iterative experimentation, and witness how artistic exploration improves every iteration.
This is especially poignant as it means that everything is described in a detailed and in-depth manner, ensuring progress in the course.
- Transformative Learning: AI art generators as a conduit for comprehensive Language Learning.
- Creative Uniqueness: The ability to create unique expressions that build on existing artistic concepts.
- Deep Comprehension: Ensuring users learn the content through interactive visual feedback.
- Dynamic Improvement: Witnessing how every artistic attempt improves every facet of AI understanding.
- In-Depth Exploration: Encouraging detailed and in-depth exploration that propels progress in artistic endeavors.
AI as Innovation Catalyst
Artificial intelligence is a potent catalyst for innovation, dramatically enhancing the creative process by employing sophisticated algorithms such as neural networks, Generative Adversarial Networks (GANs), and Long Short-Term Memory networks (LSTMs).
These technologies facilitate language learning, interpreting prompts with deft understanding and generating art concepts with minimal questions. The reliable infrastructure provided by cloud computing supports these AI systems, ensuring that the creative challenge is challenging enough to stretch the imagination while enabling easier maintenance of progress.
The grading and fast response of the AI tools have made it much easier for creators to iterate and improve. Users are often impressed with the mix of responsiveness and helpfulness without human intervention.
The journey with AI in art is worth the endeavor, unlocking unprecedented avenues for innovation.
FAQs
What does “100% Neural” mean in the context of AI art generation?
100% Neural” suggests that the art generation is solely driven by neural networks, emphasizing the exclusive use of artificial intelligence techniques, such as Generative Adversarial Networks (GANs) or other neural models.
How can I master AI art generator techniques as a beginner?
To master AI art generator techniques, start by learning the basics of neural networks, particularly GANs. Explore frameworks like TensorFlow or PyTorch and work through tutorials and hands-on projects to gain practical experience.
What distinguishes the AI art generation from traditional art techniques?
AI art generation involves algorithms and neural networks learning patterns from data to create new artworks, whereas traditional art techniques rely on human skill and creativity. AI art often explores novel styles and patterns.
Can AI art generator techniques replicate specific art styles?
AI art generators can be trained to replicate specific art styles through techniques like style transfer. By exposing the model to examples of a particular style, it can learn to generate art that reflects those stylistic elements.
What role do datasets play in mastering AI art generator techniques?
Datasets are crucial for training AI art generators. A diverse and well-curated dataset provides the model with a broad range of examples, enabling it to learn various styles, patterns, and features.