Digital Art Transformed: 11 Neural Styles
In the dynamic intersection of technology and art, neural networks have emerged as a transformative force, redefining the boundaries of digital creativity.
The evolution of these sophisticated models has given rise to a myriad of neural network styles, each with its unique approach to modifying and generating digital artworks. As we survey 11 of these groundbreaking styles, we encounter DeepDream’s phantasmagoric landscapes, the adversarial interplay within Generative Adversarial Networks, and the nuanced brushstrokes of style transfer techniques.
These neural architectures are not merely tools but collaborators that expand the artist’s palette in unprecedented ways, blurring the line between the creator and the created. Considering their impact on the digital art scene, one must ponder the implications of such advancements—how they challenge our traditional perceptions of artistry, authorship, and the creative process itself.
The question remains how these neural network styles will continue evolving and what new artistic expression forms will engender.
Key Takeaways
- Neural network styles in digital art, such as DeepDream, fusion technology, and art, transform photographs into extraordinary digital canvases and enhance imperceptible patterns.
- Generative Adversarial Networks (GANs) revolutionize digital art creation by enabling the generation of complex and original imagery, pushing aesthetic boundaries beyond human craftsmanship.
- Ethical considerations arise with GANs in art creation, including authorship, copyright, deepfake misuse, privacy violations, and ambiguity in ownership of AI-generated art.
- Style transfer techniques, like the Neural Algorithm of Artistic Style, revolutionize digital image manipulation by preserving content features and applying style features using a Gram matrix, with the quality and speed influenced by model architecture.
DeepDream’s Surreal Imagery
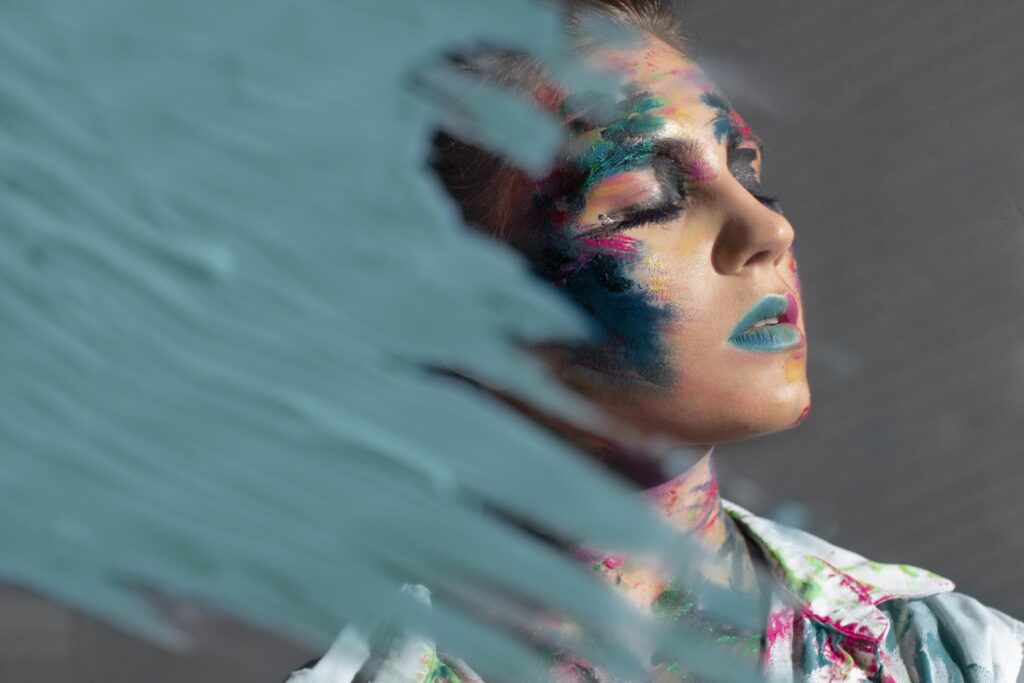
Emerging from the complex layers of Convolutional Neural Networks, DeepDream’s Surreal Imagery represents an intriguing fusion of technology and art, transforming mundane photographs into extraordinary digital canvases through pattern enhancement and feature amplification.
At the core of this process lies a deep neural network that meticulously analyzes an image’s intricate patterns, iteratively adjusting pixel values to maximize the activation of specific features within the network’s layers. This computational art form leverages the inherent capacity of convolutional neural networks to detect and accentuate patterns often invisible to the human eye, thereby crafting a visually artistic reinterpretation of the original input.
DeepDream’s methodology is effectively rooted in the principles of image processing techniques, utilizing backpropagation to project the neural network’s learned representations back onto the visual space. The result is an artistic distortion, a neural reinterpretation filled with enhanced textures and surreal motifs that evoke a dream-like quality.
This style of imagery not only showcases the artistic potential embedded within deep learning models but highlights the complex interplay between neural feature extraction and creative expression.
Generative Adversarial Networks
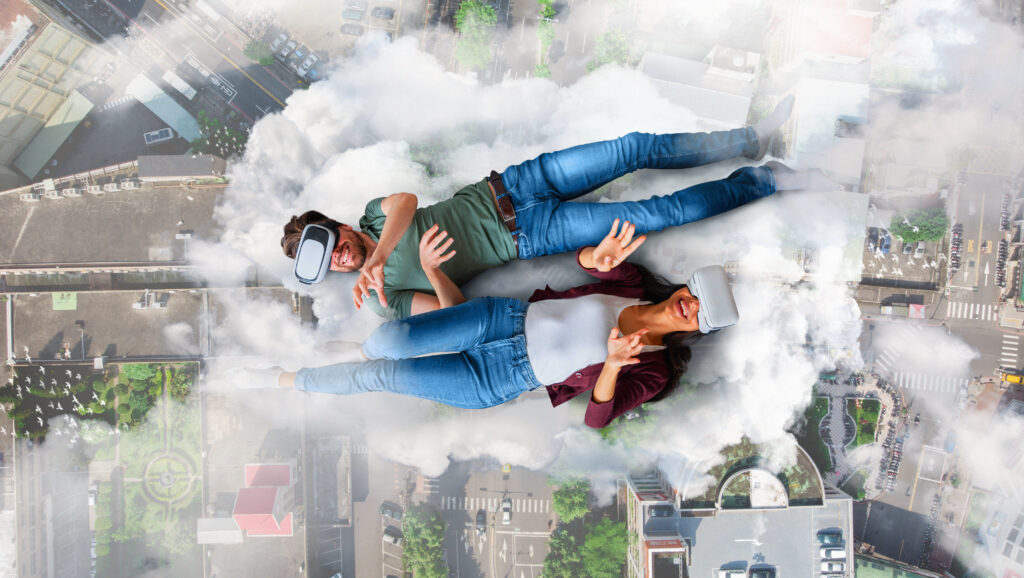
Generative Adversarial Networks (GANs) have revolutionized digital art creation by facilitating the generation of novel and complex imagery that pushes aesthetic boundaries beyond conventional human craftsmanship.
The interplay between the generator and discriminator models within GANs enables the production of high-fidelity art pieces. It raises the bar for what can be considered original or derivative in the artistic landscape.
However, the capacity of GANs to replicate and innovate upon existing styles brings forth ethical considerations regarding authorship, authenticity, and the potential for copyright infringement in the digital art sphere.
GANs in Art Creation
Harnessing the power of Generative Adversarial Networks (GANs), artists and technologists are revolutionizing digital art by producing novel and original works that push the boundaries of traditional creativity. Building on the foundational work of Gatys et al. in ‘Neural Style Transfer: Everything You Need to Know,’ these networks utilize Convolutional Neural Networks to facilitate Arbitrary Style Transfer.
By implementing Style Transfer models that rely on Deep Learning, GANs can synthesize images that quantify and apply the ‘Algorithm of Artistic Style’ to generate aesthetically compelling results. This process involves a delicate balance of content loss and style loss, guided by the ‘Neural Algorithm of Artistic Style,‘ a cornerstone in the intersection of artificial intelligence and art.
- Algorithm of Artistic Style: A framework that deciphers and applies distinct artistic nuances.
- Arbitrary Style Transfer: The ability of GANs to blend and morph various styles in unforeseen ways.
- Content Loss and Style Loss: Metrics that guide the preservation of subject matter while infusing style.
Evolving Aesthetic Boundaries
As the digital art landscape continues to expand, Generative Adversarial Networks (GANs) are redefining the concept of aesthetic boundaries by facilitating the creation of unprecedented and complex artistic expressions.
Aspect | Traditional Art | GAN-Generated Art |
---|---|---|
Style Representation | Fixed artistic styles | Evolving aesthetic boundaries |
Inspiration Sources | Human artists (e.g., Vincent van Gogh, Katsushika Hokusai) | Diverse content and style representations |
Creation Process | Manual, artist-driven | Adversarial training between generator and discriminator |
Outcome | Static visual pieces | Dynamic, unique visual expressions |
Through neural style transfer, GANs utilize a pre-trained Convolutional Neural Network to analyze and replicate the intricate nuances of content and style representations from renowned artists like Vincent van Gogh, Katsushika Hokusai, and Pablo Picasso. Yet, instead of merely aiming to transfer style, GANs push the envelope, generating art with a unique visual vocabulary that continuously transforms, thus perpetually evolving aesthetic boundaries.
Ethical Implications
The advent of Generative Adversarial Networks (GANs) has ushered in a new wave of ethical dilemmas. Their capacity to generate realistic imagery blurs the lines between authenticity and fabrication. Neural Style Transfer, a technique involving deep neural networks, exemplifies this by merging the content image with a style image, giving rise to a uniquely generated image. This synthesis, however, raises significant ethical implications.
- Deepfake Misuse: GANs can fabricate credible media, undermining trust.
- Privacy Violations: Generating images of individuals without consent.
- Intellectual Property Concerns: Ambiguity in the ownership of AI-generated art.
These technologies necessitate a rigorous analysis of the Style Transfer using Content Loss and Style Loss within the Total Loss function. This analysis ensures authenticity while mitigating ethical risks.
Variational Autoencoders in Art
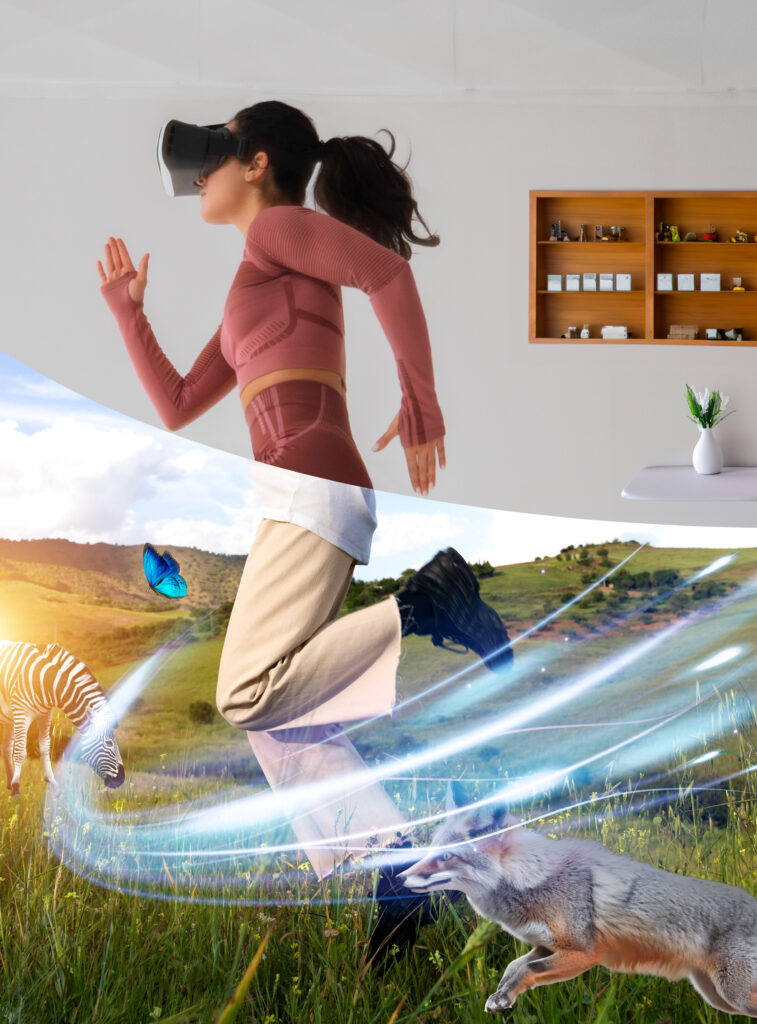
Variational Autoencoders, sophisticated tools in computational creativity, have opened new avenues for artists by enabling the generation of novel and intricate visual pieces that push the boundaries of digital art. Through their encoder-decoder architecture, these generative models analyze and reconstruct images, capturing the essence of artistic styles in the process.
The encoder part of a VAE compresses input images into a condensed representation in latent space, which the decoder then uses to reconstruct the original image or to generate new, varied outputs.
By employing convolutional neural network (CNN) layers, VAEs efficiently produce feature maps that represent abstract aspects of images. This capability is especially crucial when dealing with style images rich in textures and patterns. VAEs can be trained on a dataset of artworks to learn the probability distribution of different art styles. Introducing randomness in the latent space ensures the generation of unique artistic expressions with arbitrary styles.
Additionally, VAEs can be utilized alongside Neural Style Transfer techniques. While Neural Style Transfer is everything about extracting content from one image and fusing it with the style of another, VAEs can further the exploration of digital art by allowing the transformation of content and style into new, unpredictable creations. This synergy empowers artists to experiment with pre-trained models and manipulate visual elements in previously inconceivable ways, leading to an ever-expanding realm of digital artistry.
Style Transfer Techniques
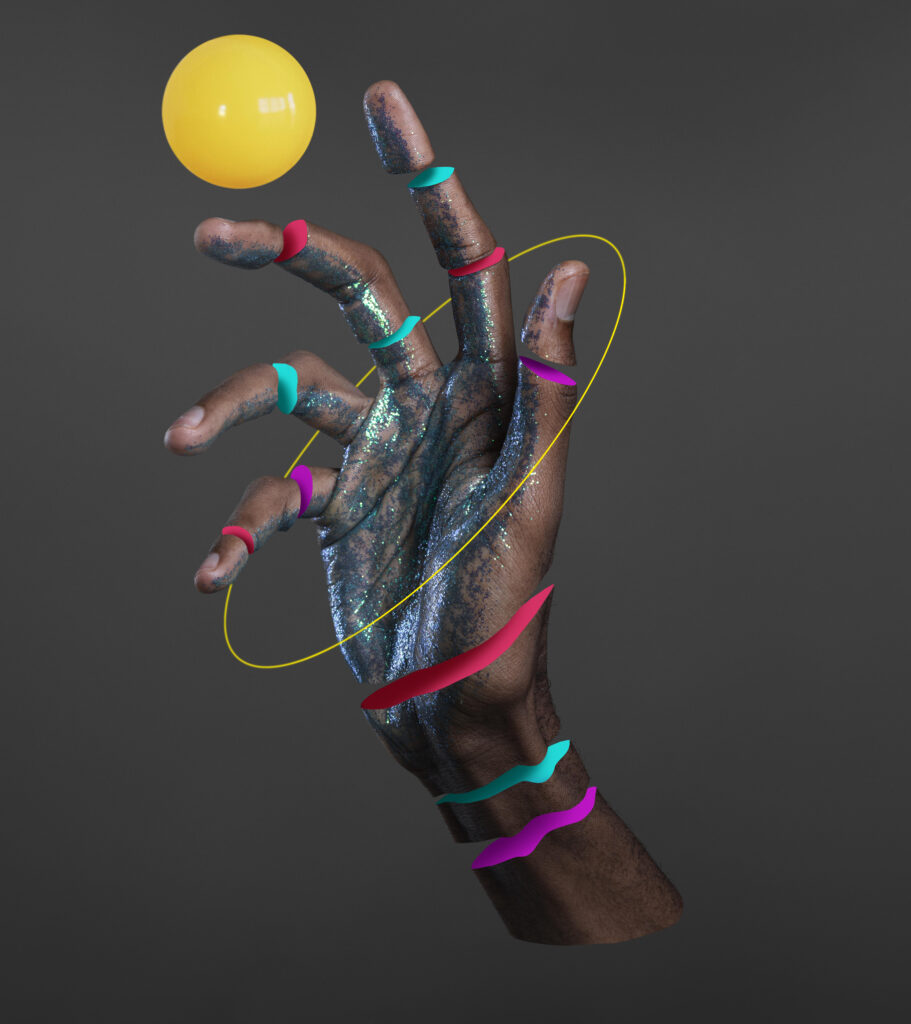
Harnessing the power of deep learning, style transfer techniques have revolutionized how digital images are manipulated, enabling the seamless fusion of artistic styles into various forms of content. At the heart of this technique is the Neural Algorithm of Artistic Style, which leverages a neural network to separate and recombine the content and style of images. This process involves defining and optimizing two key components: content loss and style loss. Content loss ensures the ‘content features’ of the target image remain intact, while style loss uses a ‘Gram matrix’ to measure and apply the ‘style features’ from the source style.
Model architecture plays a crucial role in the efficiency of style transfer algorithms, impacting quality and speed. For instance, Real-Time Style Transfer models use a streamlined network structure to apply a single style almost instantaneously. Meanwhile, more complex architectures allow for arbitrary style transfer, requiring significant computational resources but offering greater versatility.
To engage the audience with the technical aspects of style transfer, consider the following elements:
- The intricate balance between content loss and style loss to achieve high-quality transfer results.
- The role of the Gram matrix in capturing and applying complex style patterns.
- The advancements in Model architecture enable Real-Time Style Transfer and arbitrary style application.
Recurrent Neural Networks’ Contribution
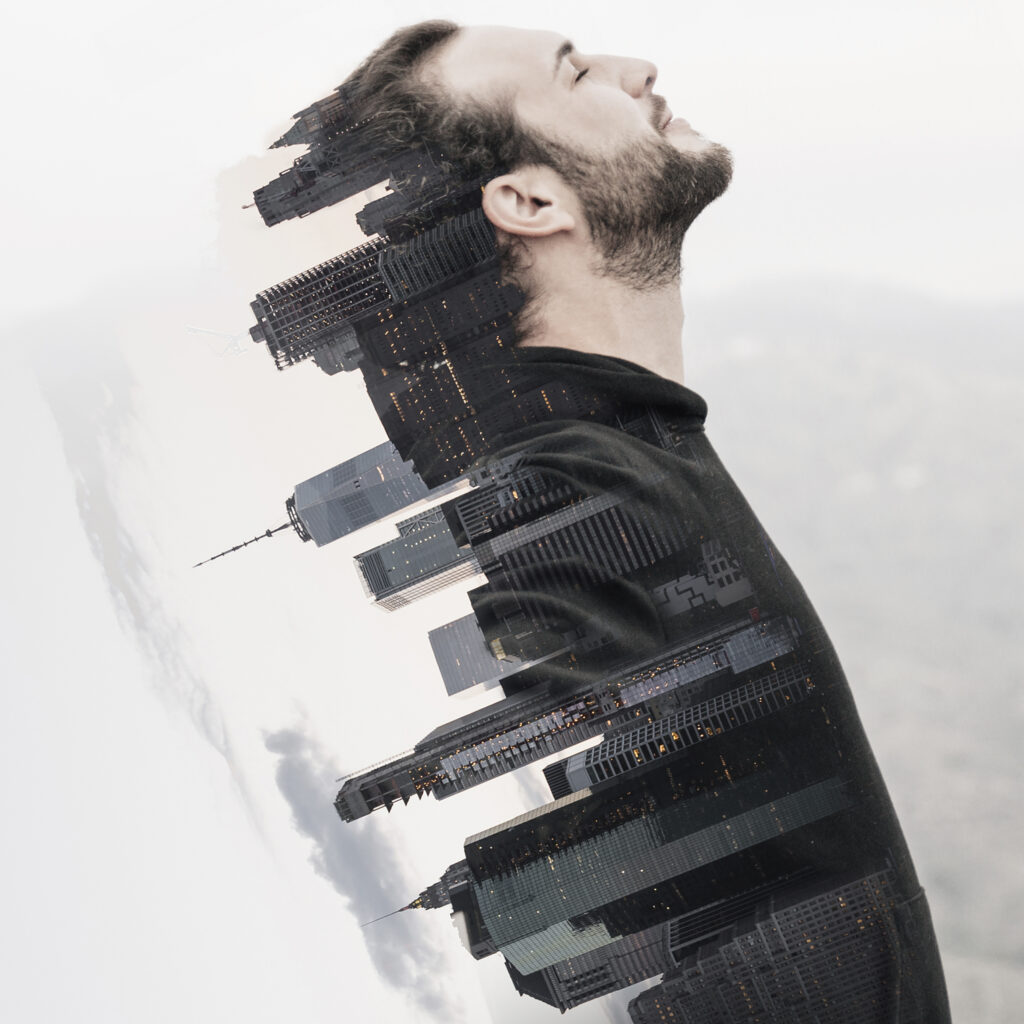
Recurrent Neural Networks (RNNs) stand out in the digital art landscape for their ability to encapsulate temporal dynamics within artworks, thus capturing the essence of motion and the passage of time. These networks elevate sequential artistry by maintaining contextual coherence across frames, facilitating the portrayal of narrative-driven sequences with enhanced fluidity.
Moreover, RNNs are instrumental in text-to-image applications, where the conversion of narrative text into rich, sequential visual content is paramount to the storytelling aspect of digital art.
Capturing Temporal Dynamics
In digital art transformation, Recurrent Neural Networks (RNNs) play a pivotal role in capturing and replicating the nuanced temporal dynamics inherent to evolving artistic styles. These deep-learning models excel in processing and generating sequences. This capability is crucial for the Style Transfer domain, where the temporal aspect of art can be encoded and transferred.
RNNs’ ability to model sequential data is leveraged to enhance the depth of Style Transfer algorithms, ensuring that the Output Image reflects temporal artistic progressions.
Their memory component aids in applying Loss functions, such as Content Loss and Perceptual Losses for Real-Time Style Transfer, considering the context and sequence of the artwork.
Incorporating Gram matrix computations within RNN frameworks allows for a sophisticated representation of style features, translating to more dynamically evolved Computer Vision applications powered by Pre-trained CNNs.
Enhanced Sequential Artistry
Building upon the capabilities of Recurrent Neural Networks in capturing temporal dynamics, Enhanced Sequential Artistry emerges as a compelling application in digital art, enabling the generation of images that unfold in a narrative sequence.
By harnessing deep learning techniques, RNNs analyze and replicate complex patterns in data, which translates to sequential image generation that reflects a cohesive story in the context of digital art.
The process often involves style transfer, where the stylistic elements of one image are applied to another. Using a Gram matrix to capture style information from a reference image, such as a painting by Vincent van Gogh, RNNs can minimize content loss while transferring this image style onto a newly generated sequence.
This facilitates a third image that can fluidly blend the content and style information, creating a dynamic visual narrative consistent with the sequential art form.
Text-to-Image Applications
Harnessing the sequential processing power of Recurrent Neural Networks, Text-to-Image applications are revolutionizing the way digital art translates textual descriptions into complex visual representations.
Deep Learning frameworks enable RNNs to iteratively refine images through a process that mirrors the basic principle of Style Transfer, intertwining the style of an image with textual cues to generate content images. This synergistic application of Style Transfer and Super-Resolution techniques within RNN architectures facilitates a nuanced image content Transfer process underpinned by the intricate workings of Style Networks.
- Content Loss Minimization: RNNs are trained to minimize content loss, ensuring that generated images faithfully represent the text’s intent.
- Sequential Context Capture: The network captures linguistic sequences to preserve context, enabling more coherent and detailed imagery.
- Adaptive Style Learning: Style Networks within RNNs learn and apply artistic styles dynamically, enriching the visual output based on textual descriptions.
Transformer Models’ Visual Feats
Transformer models have revolutionized the realm of digital art by applying their self-attention mechanisms to master visual dependencies, enhancing tasks such as image style transfer with unprecedented efficiency and accuracy. These models, grounded in the principles of Deep Learning, leverage Neural Networks to render the subtleties of artistic styles onto target images, maintaining a delicate balance between the original image’s content and the applied aesthetic.
The style transfer model encodes the original image and a chosen style reference—often Famous Paintings like those by Vincent van Gogh—into deep feature representations. The model’s hidden layers then engage in a sophisticated dance, directed by the Transfer: Everything You Need principle, optimizing the Content Loss to ensure the core elements of the original image persist while the style characteristics are effectively overlaid.
A critical component in this intricate process is the Gram matrix, a mathematical construct that captures the texture information from the style reference. By calculating the Gram matrix at each hidden layer, the network learns to replicate the brushstrokes and color patterns that define the style of artists like Van Gogh.
This intricate interplay between content and style, executed with the computational prowess of transformer models, is setting a new standard for digital artistic expression.
Capsule Networks and Digital Creativity
Capsule Networks represent a paradigm shift in digital artistry, offering a nuanced understanding of spatial hierarchies that traditional convolutional neural networks often struggle to capture. Embedded within Deep Learning, these networks are redefining the possibilities in transforming digital art, allowing artists and algorithms to generate new, complex compositions with unprecedented detail.
Using a constellation of intricately designed capsules, these networks can recognize and retain visual data’s positional and relational features. This capability is essential in tasks such as new image content transfer—where the goal is not merely to apply styles but to understand the underlying structures of the manipulated content. As a result, capsule networks have become instrumental in advancing digital creativity.
To further draw the audience into the technical brilliance of capsule networks, consider the following points:
- Capsule networks encode multiple properties of an image, leading to a richer and more accurate representation of styles.
- They significantly enhance the quality of image content transfer by preserving the coherence of spatial relationships.
- Their robust feature extraction methods open new avenues for creating digitally creative content that is contextually complex and visually striking.
In leveraging the capabilities of capsule networks, the digital art landscape is witnessing a transformative era where the interplay of algorithmic precision and artistic vision produces groundbreaking works of art.
FAQs
What are neural network styles in the context of digital art?
Neural network styles refer to the application of deep neural networks, particularly style transfer algorithms, to transform the visual style of digital art. These networks can take an input image and apply the artistic style of another image to create a new, stylized output.
How do neural network styles work in digital art?
Neural network style transfer involves pre-trained convolutional neural networks (CNNs) to separate and recombine content and style from two images. The content of an input image is preserved, while the artistic style of a reference image is applied to create a new, stylized result.
Which neural network architectures are commonly used for style transfer in digital art?
Convolutional Neural Networks (CNNs) are commonly used for style transfer in digital art. Specific architectures like VGG-19 and ResNet are often employed for their ability to capture complex features and textures, which are crucial for preserving content and applying styles effectively.
Can neural network styles be applied to various forms of digital art?
Yes, neural network styles can be applied to various digital art forms, including images, illustrations, paintings, and videos. The techniques are versatile and can be adapted to different types of visual content.
Are there specific tools or software for applying neural network styles to digital art?
Yes, several tools and software facilitate the application of neural network styles. Some popular ones include DeepArt, NeuralStyler, and neural network style transfer implementations in deep learning frameworks like TensorFlow and PyTorch.