AI Art Revolution: Top Neural Networks
In artificial intelligence, the emergence of top-tier neural networks has catalyzed a transformative shift in the landscape of art generation. These sophisticated algorithms, such as OpenAI’s DALL-E 2 and Google’s DeepDream, have demonstrated an astonishing capability to produce visually compelling artwork and raised profound questions about the nature of creativity and the future role of human artists.
As we witness a burgeoning gallery of AI-generated art, which ranges from the surreal to the hyperrealistic, exploring the underlying technologies that make such innovation possible is essential. From the intricacies of Generative Adversarial Networks to the subtleties of Transformer models, each neural network architecture contributes uniquely to the tapestry of AI art.
Yet, as we stand at this crossroad, we must consider the ethical and conceptual implications of this digital renaissance, engaging in a nuanced conversation about integrating artificial intelligence into the creative domain.
Key Takeaways
- GANs and VAEs are two critical neural networks revolutionizing AI art generation.
- Ethical concerns regarding the authenticity and responsible use of AI-generated content have emerged.
- VAEs optimize creative exploration within the latent space, enhancing image generation.
- With their self-attention mechanisms, transformer models have revolutionized natural language understanding and generation in the context of AI art generation.
Emergence of Generative Adversarial Networks
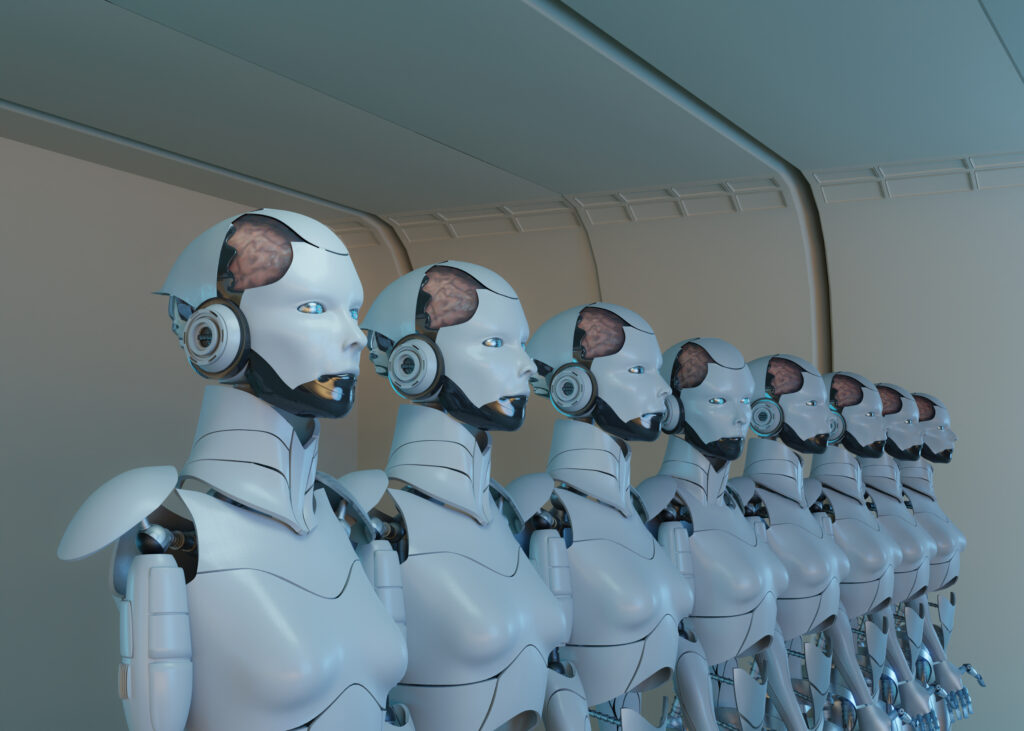
The advent of Generative Adversarial Networks (GANs) has marked a transformative era in artificial intelligence, particularly in synthetic art generation. GANs, a class of AI constituted by two competing neural networks—the generator and the discriminator—exemplify the strides made in deep learning algorithms. The generator produces art while the discriminator evaluates it, instigating a dynamic where both networks iteratively enhance their functions. This symbiotic rivalry fosters an environment where AI can generate unique, intricate pieces that often parallel the creativity typically ascribed to human artists.
The implications of GANs in AI art generation are profound, vastly expanding the possibilities for artists and designers. By harnessing GANs, creators can explore novel aesthetic territories unbridled by the constraints of traditional mediums or personal skill limitations. Moreover, GANs have transcended the boundaries of visual art, influencing domains such as fashion, advertising, and even natural language processing.
Yet, this innovation is subject to controversy. Ethical concerns have surfaced over the authenticity of AI-generated content and the responsible stewardship of such machine learning algorithms. The dialogue surrounding GANs thus extends beyond technical marvels to encompass a broader discourse on the intersection of AI and human creativity.
Breakthroughs With Variational Autoencoders
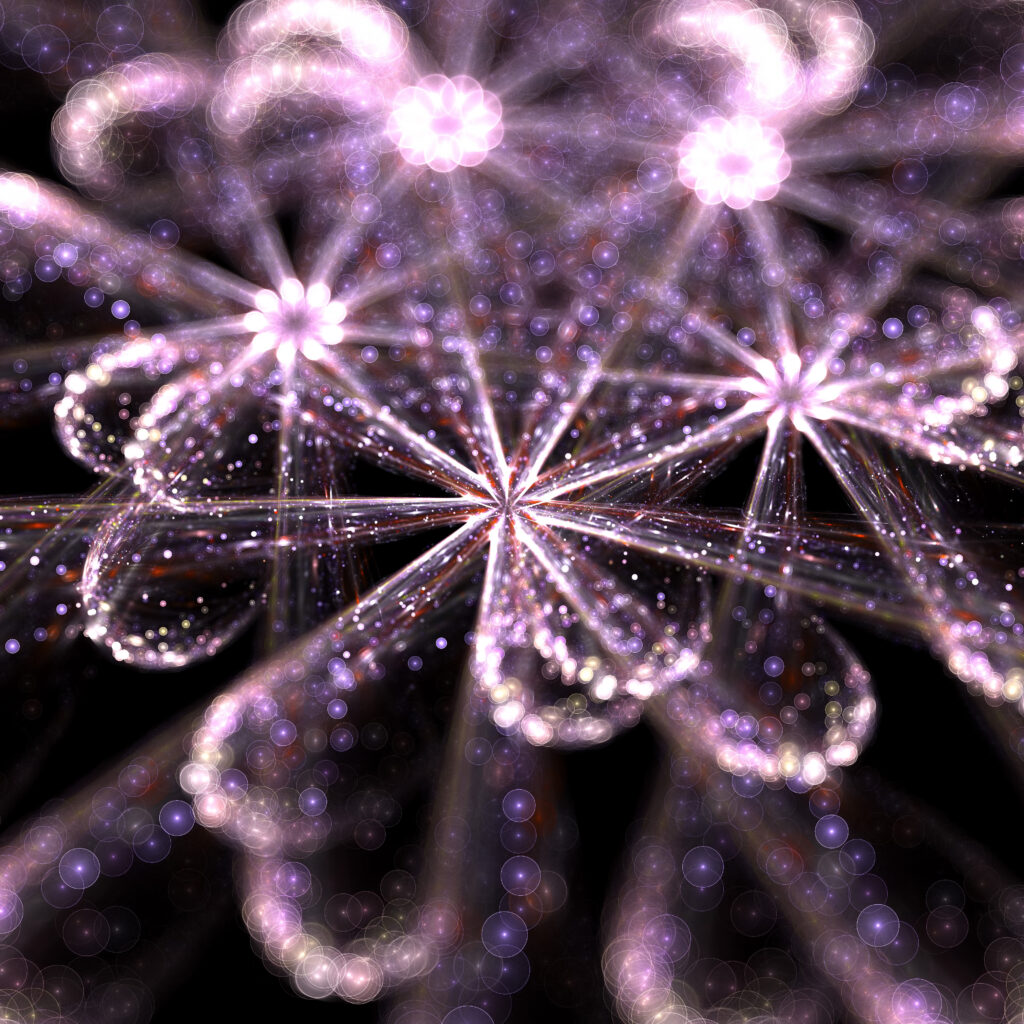
The advent of Variational Autoencoder (VAE) architectures has significantly refined the image generation process, enabling the production of varied and complex visual content. By optimizing the creative exploration within the latent space, VAEs facilitate the generation of unique and intricate patterns applicable across various domains, from medical imaging to artistic creation.
These breakthroughs underscore the transformative potential of VAEs in augmenting creative processes and advancing the capabilities of AI-driven art generation.
Enhancing Image Generation
In artificial intelligence, Variational Autoencoders (VAEs) have emerged as a transformative tool in enhancing image generation, offering unprecedented realism and detail. These generative neural networks use machine learning algorithms to:
- Learn Complex Patterns: VAEs analyze vast datasets to discern intricate patterns, creating new images that reflect learned textures and details.
- Improve Image Quality: VAEs generate stunning visuals with enhanced clarity and resolution by refining output fidelity.
- Increase Diversity: These art generators introduce variability, ensuring each AI-generated image is unique and distinct.
- Enhance Controllability: VAEs allow precise manipulation of image features, allowing artists to craft specific attributes within their AI image generators.
VAEs are revolutionizing how we create and perceive art in the digital age through these capabilities.
Variational Autoencoder Architectures
Building on the strengths of Variational Autoencoders in enhancing image generation, the underlying architectures have paved the way for groundbreaking developments in AI-driven art creation. These VAEs, integral to advancing generative AI, utilize sophisticated algorithms to capture and reconstitute the vast creative possibilities inherent in art. Their capacity for neural style transfer and generating high-quality images has been transformative. As AI art generators become more prevalent, the role of VAEs is increasingly recognized for their contribution to artificial intelligence’s creative frontier.
Feature | Impact on AI Art | Technical Aspect |
---|---|---|
Generative Capability | Diverse visual content | High-dimensional data modeling |
Learning Efficiency | Enhanced quality and variety | Complex data distribution learning |
Neural Style Transfer | Unique artistic expressions | Encoding and decoding complex inputs |
Creative Latent Space Exploitation
Harnessing the power of variational autoencoders, creative latent space exploitation emerges as a pivotal breakthrough in AI-generated art, enabling unprecedented manipulation and synthesis of artistic elements. This technique has revolutionized the capabilities of art generators, allowing them to:
- Seamlessly blend diverse artistic styles and visual elements, fostering a fusion of human creativity with AI algorithms.
- Analyze vast data to generate images pushing conventional art forms’ boundaries.
- Offer artists and enthusiasts tools to explore and expand the creative potential of neural networks in art generation.
- Produce personalized artistic outputs that reflect the intricacies and nuances of individual preferences and styles.
Through these advancements, variational autoencoders are redefining the landscape of AI art generators, creating a symbiosis between technology and artistic expression.
Transformative Impact of Transformer Models
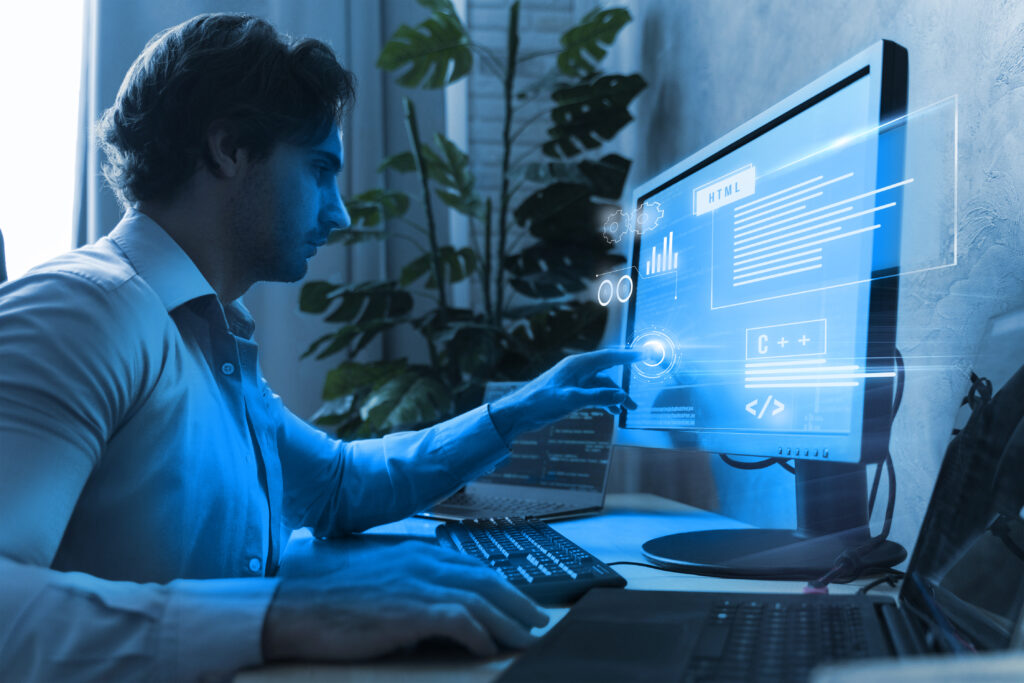
Transformer models, characterized by their self-attention mechanisms, have catalyzed a paradigm shift in natural language understanding and generation. Their architecture not only enhances the creative potential of AI by producing contextually rich text but also underpins a multitude of real-world applications, from machine translation to content creation.
This discussion will elucidate the underlying principles of transformer models, examine their role in amplifying creativity within AI art generation, and survey their practical deployment across various industries.
Transformer Models Explained
At the heart of recent advancements in artificial intelligence, specifically in natural language processing, lie transformer models—a class of neural networks that leverage attention mechanisms to enhance the understanding and generation of human language dramatically. These models have altered the landscape of what’s possible in AI, providing a foundation for systems that can analyze vast amounts of textual data with unprecedented accuracy and speed.
Consider the following key points about transformer models:
- Transformer models excel at capturing long-range dependencies in data.
- Their parallel processing capabilities lead to efficient learning and better performance on complex tasks.
- They have revolutionized the creative process in AI by enabling algorithms to create more nuanced and contextually relevant outputs.
- Continuous refinement of these models ensures improvements in AI models used for art creation and beyond.
Enhanced Creative Potential
Building upon the foundation set by their ability to comprehend and produce human language, transformer models have propelled AI into a new era of artistic creation, where synthesizing high-quality, diverse, and original artwork is not just possible but increasingly commonplace.
With the advent of AI art generators available today, artists are empowered to push the boundaries of visual expression, crafting stunning masterpieces that are both unique and captivating. These transformer-based systems facilitate experimenting with various art styles, enabling the emergence of true creativity.
Moreover, the customization options inherent to these tools offer new possibilities for artists who wish to use an AI collaborator. As a result, transformer models are redefining the landscape of art generation, merging deep learning algorithms with the nuanced domain of human aesthetics.
Real-world Applications Explored
Looking into the real-world applications of transformer models reveals a transformative impact across various sectors. These include linguistics, art, and music, where their ability to generate human-like content is being harnessed to innovate and streamline creative processes.
- Content Creation: Transformer models act as advanced art generators, synthesizing stunning and unique artworks that blend styles and genres. This pushes the boundaries of the world of AI art.
- Linguistic Analysis: These neural networks facilitate the creation of new linguistic models that understand and generate complex language structures. They leverage vast amounts of data to achieve this.
- User Interaction: Transformer models allow users to input prompts and receive personalized images based on specifications. This democratizes art creation and gives users more control over the final output.
- Ethical Framework: The deployment of transformer models necessitates rigorous ethical considerations. These considerations revolve around their transparency and the implications of AI-generated content.
Advancements in Recurrent Neural Networks
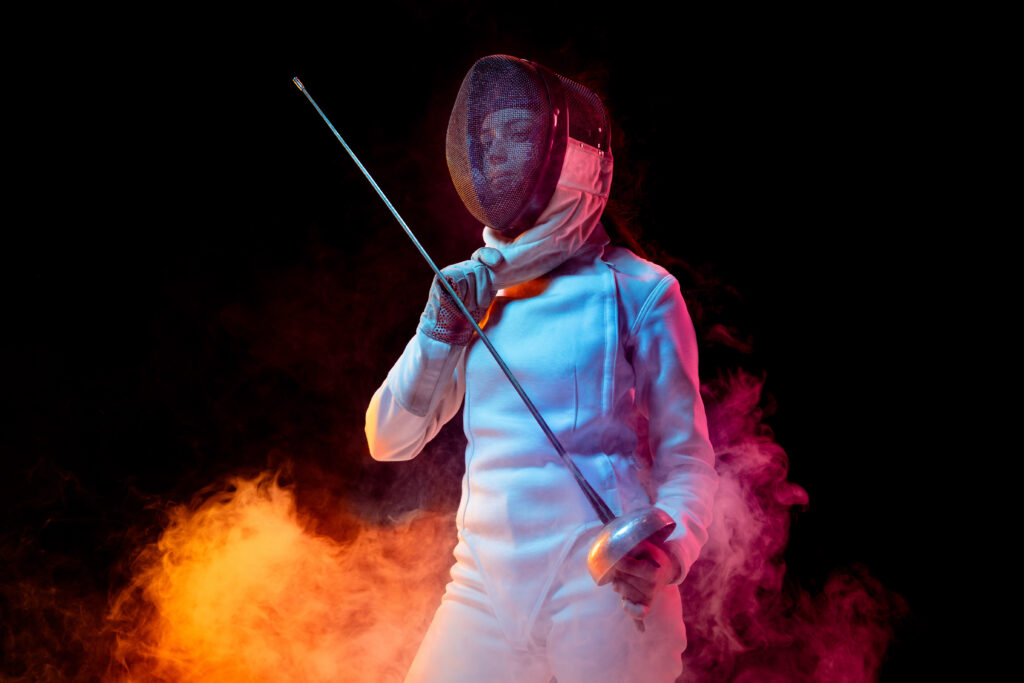
Significant strides in the evolution of Recurrent Neural Networks (RNNs) have been made through the introduction of advanced architectures like Long Short-Term Memory (LSTM) and Gated Recurrent Unit (GRU), which effectively overcome the challenges of long-term dependency modeling inherent in earlier RNN designs. These innovations have substantially enhanced the capabilities of neural networks in processing and predicting sequential data with high precision.
The integration of LSTM and GRU has been particularly impactful in AI art generators, enabling these tools to understand better and replicate complex sequences of artistic styles. This results in art generators that offer more nuanced and varied outputs, closely mirroring the intricacies of human-created art. Artists and users leveraging these AI tools can now create with an unprecedented level of detail and coherence over extended sequences, pushing the boundaries of automated art generation.
Adding attention mechanisms to RNNs has further refined their functionality, allowing networks to selectively concentrate on salient features of the input data, thereby improving the relevance and quality of the generated art. These advancements position RNNs as indispensable tools in the continuously evolving landscape of AI-driven artistry, where the ability to generate art that resonates with human aesthetics is increasingly valued.
Evolution of Convolutional Neural Networks
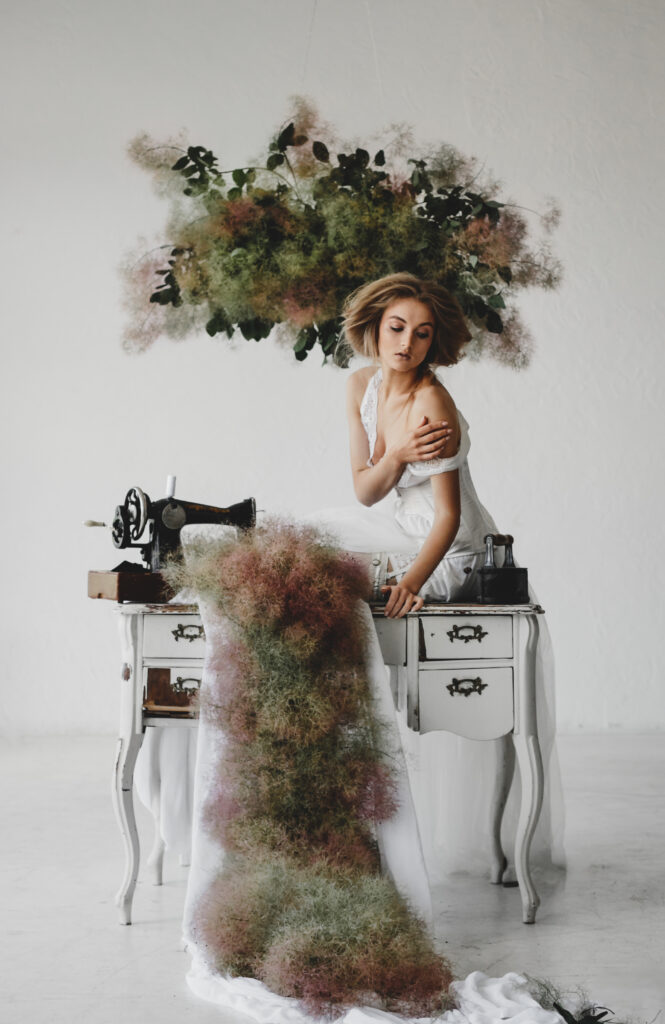
While Recurrent Neural Networks have reshaped the landscape of sequential data interpretation and generation, Convolutional Neural Networks (CNNs) have concurrently advanced to redefine how machines understand and process visual information. The evolution of CNNs has been pivotal in the development of AI, particularly in the domain of AI art generation, where these neural networks have become a cornerstone.
- Enhanced Visual Parsing: CNNs have been refined to intricately parse visual data, allowing for more extraordinary nuances in recognizing and classifying various artistic styles. This has been transformative for art generators that can now emulate complex art forms with higher fidelity.
- Efficiency and Accuracy: Progress in CNN architectures has significantly increased accuracy while reducing computational costs, enabling art creation more efficiently, which is crucial for artists and art enthusiasts leveraging AI tools.
- Diverse Artistic Tools: The range of tools powered by CNNs has expanded, allowing users to unleash their creativity through platforms where an artist can use nuanced controls to create unique pieces.
- Accessibility and Innovation: As CNNs evolve, they democratize the ability to generate art, fostering an environment where innovative artistic expression is accessible to a broader audience, thus continually enriching the creative landscape.
Innovation With Deep Learning Compositing
Deep learning compositing has emerged as a groundbreaking technique that leverages neural networks to forge composite imagery with a level of detail and realism once thought impossible in digital art and visual effects. By harnessing the computational power of deep learning algorithms, this method has redefined the capabilities of art generators, particularly AI art generators, to innovate and create original pieces that are both visually appealing and technically sophisticated.
The technique’s analytical prowess lies in its ability to combine and manipulate visual elements from multiple sources, integrating them into a cohesive whole that belies the complexity of its formation. Neural networks analyze the textural, lighting, and color consistencies between disparate elements to ensure a seamless blend, paving the way for a new digital art era.
This innovation not only empowers artists with tools to craft a diverse range of visual narratives but also enhances the efficiency of producing complex compositions. The resultant works captivate audiences with their authenticity and artistic expression, heralding a transformative phase in the evolution of visual content creation.
Therefore, Deep learning compositing stands at the forefront of technologies, expanding the boundaries of what can be achieved in AI-generated art.
Synergy of AI and Human Creativity
Building upon the advancements in deep learning compositing, the synergy between AI and human creativity is forging a new artistic expression and innovation paradigm. Art generators have gained significant traction, providing artists with tools that leverage neural networks to analyze and reproduce many artistic styles. This technology enables artists to expand their creative repertoire to explore new horizons in art. However, as we blur the lines between AI-generated and human-made art, we encounter ethical implications that challenge our understanding of authorship and creativity.
Engage with the following key points:
- Neural Networks as Collaborative Tools: Harnessing neural networks, art generators provide new avenues for artists to explore and infuse their work with computational creativity.
- Enhancing Human Creativity: These systems are not replacements but tools that augment the artist’s capability to create, offering a blend of precision and personal touch.
- Ethical Considerations: As AI becomes more involved in the creative process, it is imperative to strike a balance that respects the integrity of human artistry.
- Cultural Repercussions: AI’s integration into art can reshape cultural narratives and redefine artistic boundaries.
In this analytical exploration, we consider the technical aspects of AI art generation, its impact on human creativity, and the ongoing dialogue to maintain the essence of personal touch in art.
FAQs
Can artists control the creative output of neural networks in AI art creation?
Yes, artists can control the creative output of neural networks by manipulating various parameters, adjusting input data, and guiding the training process. This control allows for the customization of AI-generated artwork.
Are there specific neural network architectures designed for artistic style transfer?
Several architectures, such as Neural Style Transfer (NST), have been developed specifically for artistic style transfer. NST models analyze the content and style of images to create visually appealing compositions.
What advancements have neural networks brought to the traditional art creation process?
Neural networks have brought advancements such as the ability to generate novel and diverse artistic styles, automate certain aspects of the creative process, and assist artists in exploring new possibilities beyond traditional techniques.
How has the AI art revolution influenced the definition of creativity in the art world?
The AI art revolution has expanded the definition of creativity by introducing collaborative processes between artists and machines. It challenges traditional notions of authorship and explores the co-creative potential of human-AI collaboration.
Can AI-generated art created with neural networks be considered authentic and valuable?
Yes, AI-generated art created with neural networks can be considered authentic and valuable. The value lies in the creativity embedded in the design of neural networks, the training process, and the unique outputs they generate.