Top Neural Networks for Art Creation
In recent years, the intersection of artificial intelligence and the arts has witnessed significant advancements, primarily facilitated by the emergence of neural networks adept at generating artwork. These sophisticated algorithms, such as DALL-E 2, Prodia, StarryAI, Bing Image Creator, and Leap AI, have garnered attention for their ability to produce diverse and intricate pieces that challenge traditional notions of creativity.
Employing machine learning techniques, substantial computational power, and extensive datasets, these neural networks have demonstrated an unprecedented capacity for image generation, leveraging diffusion models or Generative Adversarial Networks (GANs) to craft visuals that resonate with novelty and familiarity.
As we survey the landscape of AI-driven art, it becomes apparent that the implications of these technologies are profound, not only in their artistic applications but also in their potential to redefine the role of human artists. The question then arises: how are these neural networks shaping the future of art creation, and what does their evolution portend for the broader artistic community?
To explore this inquiry, one must consider the technical underpinnings, the emerging trends, and the broader societal impacts of these AI systems, which are rapidly becoming integral to the fabric of digital creativity.
Key Takeaways
- Neural networks, particularly Generative Adversarial Networks (GANs) and Convolutional Neural Networks (CNNs), play a crucial role in revolutionizing art creation.
- AI art generators like DALLE-2, PetalicaPaint, and Jasper Art push the boundaries of creative expression and enable the transformation of various artistic styles.
- Neural networks such as DALL-E 2 and Deep Dream Generator produce dreamlike digital art, while PetalicaPaint specializes in the automatic coloring of black-and-white artwork.
- The future of AI in art generation holds the potential to enhance nuanced and detailed artworks. Still, it also requires addressing ethical considerations and intellectual property concerns and fostering collaboration between artists and AI.
Pioneering Neural Networks in Art
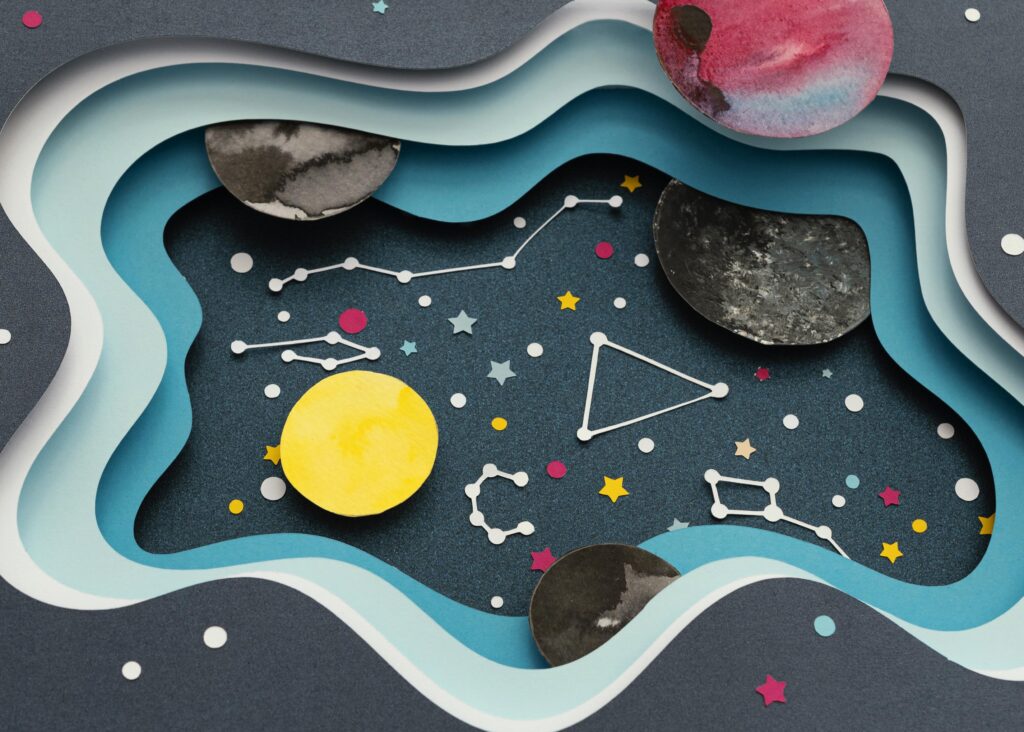
The advent of neural networks has frequently revolutionized the domain of art creation, enabling the synthesis of novel visuals through advanced computational techniques. AI art generation has emerged as a significant application of artificial intelligence (AI), leveraging neural networks to generate new forms of imagery. Generative Adversarial Networks (GANs) and Convolutional Neural Networks (CNNs) are at the forefront of this innovation, underpinned by deep learning methodologies.
GANs function through competing neural networks, with one network generating images and another evaluating them. This dynamic fosters the creation of highly detailed and credible artworks. The training data used for these AI algorithms substantially influences the output, necessitating a vast collection of training images to guide the generative process effectively.
Convolutional neural networks, instrumental in image recognition tasks, have been adapted for Generative AI, interpreting and manipulating visual data at various levels of abstraction. By processing the characteristics of the training images, these networks facilitate the production of complex and aesthetically compelling art pieces.
Platforms like DALL-E 2 and Deep Dream Generator exemplify the utilization of neural networks in art, with the former using diffusion techniques for text-to-image translations and the latter reimagining existing images through a dream-like transformation process. These pioneering applications underscore the transformative potential of neural networks in creative expression.
AI-Driven Artistic Breakthroughs
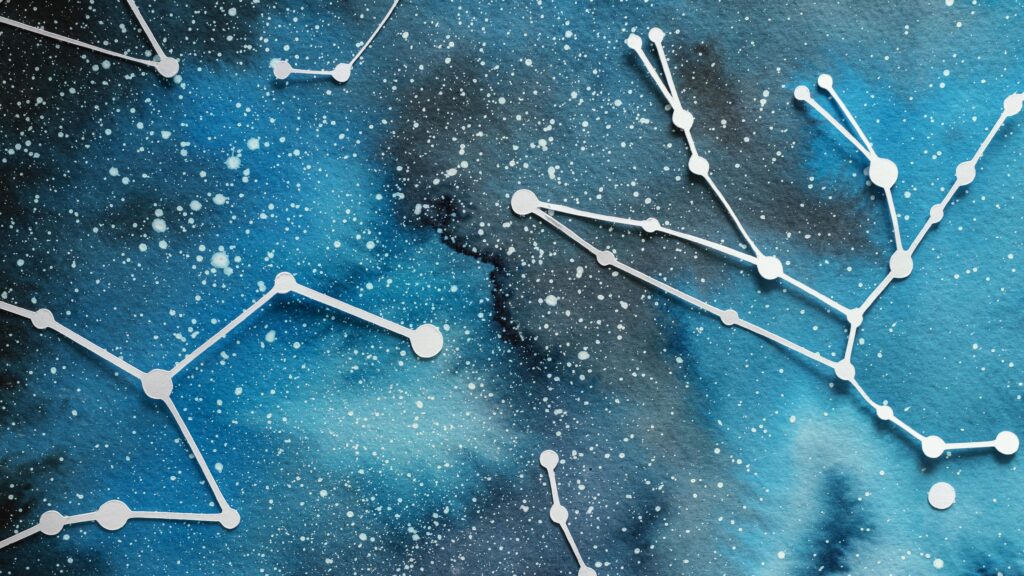
Building upon the foundational advances offered by neural networks in art, recent AI-driven artistic breakthroughs have expanded the boundaries of creative expression through innovative generators and platforms. These AI art generators harness the capabilities of generative adversarial networks and other machine learning models to create new, aesthetically compelling artworks.
For instance, text-to-image AI art generators like DALLE-2 utilize deep learning to translate descriptive language into diverse and imaginative visual content.
Some platforms focus on using artificial intelligence to create specific types of art. PetalicaPaint applies machine learning algorithms to colorize black-and-white artwork automatically, simplifying a traditionally time-consuming process. Meanwhile, generators like Jasper Art are adept at using machine learning to create unique images based on user input, generating artwork that reflects individual tastes and preferences.
Moreover, AI art generators such as DeepArt.io and Runway ML enable artists to generate new images by transforming photographs into different artistic styles or creating art with code. These networks to develop new creative outputs are not just tools for replication; they are instruments of innovation, providing an unprecedented avenue for generative art and opening up a world where the fusion of technology and creativity yields endless possibilities.
Neural Networks Shaping Digital Creativity
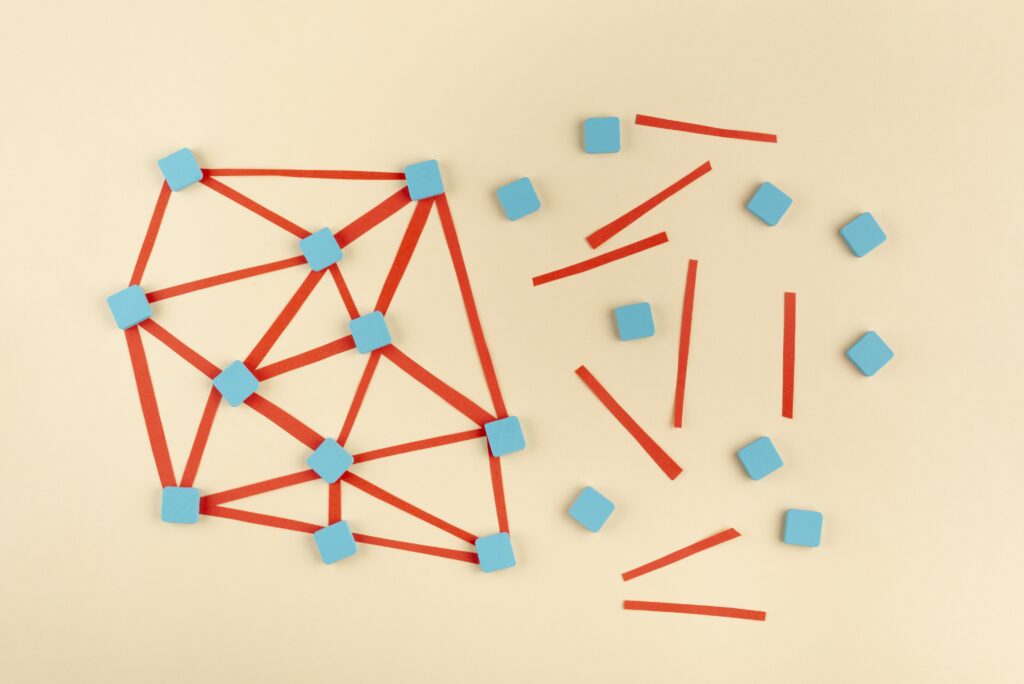
Harnessing the transformative power of neural networks, digital creativity has entered a new era of innovation where art forms are replicated and reinvented through sophisticated algorithms. AI art generators are at the forefront of this movement, utilizing artificial intelligence to push the boundaries of visual expression.
These tools facilitate an intricate training process by employing a generator network that works with a discriminator network. The networks iterate over vast datasets, learning to generate images that can mimic or transcend traditional styles.
Neural networks such as DALL-E 2 and Deep Dream Generator leverage these capabilities to produce dreamlike and surreal digital art, while ArtBreeder and AI Painter offer artists the opportunity to generate art in many styles. PetalicaPaint specializes in the automatic coloring of black-and-white artwork, showcasing the precise application of learned colorization techniques. Crayon, formerly DALL-E mini, provides tools for illustrations and high-definition visuals, reflecting the neural network’s understanding of intricate details.
Jasper Art and DALLE-2 by OpenAI epitomize the diversity achievable through neural network-based art creation. These AI-driven platforms are redefining the landscape of digital art, enabling creators to explore uncharted territories of aesthetic possibilities.
The Future of AI in Art Generation
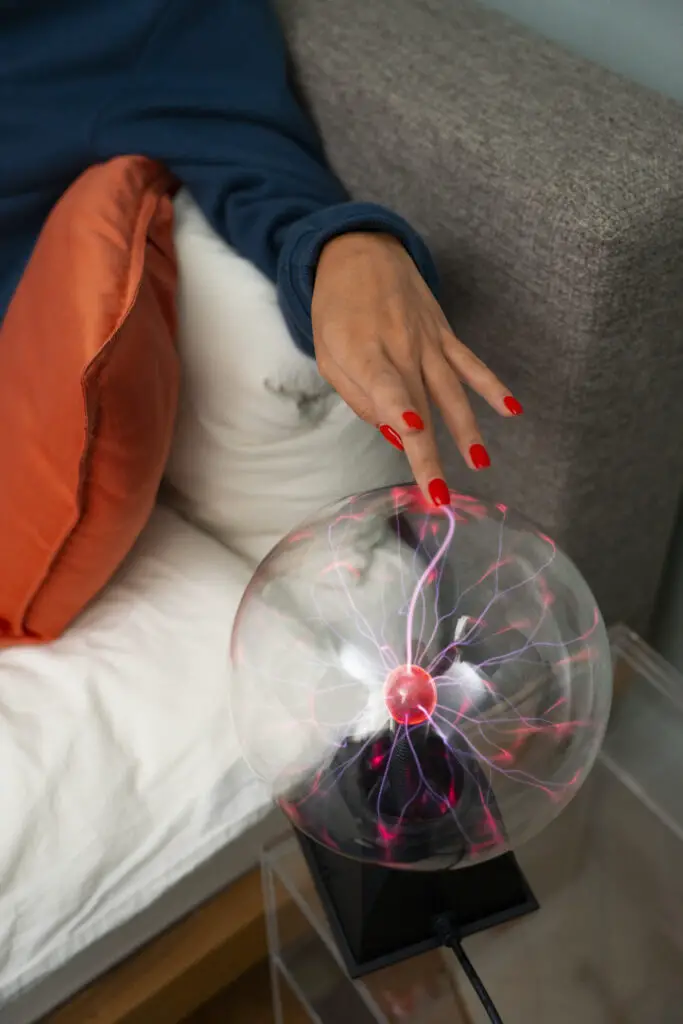
Exploring the trajectory of artificial intelligence in art generation reveals a landscape ripe for revolutionary advancements and unprecedented creative collaborations between human artists and sophisticated algorithms. As neural networks become more adept at understanding and replicating artistic styles, AI art generators are positioned to transform how art is created and consumed.
The future of AI in art generation looks to:
- Enhance the capacity of AI to generate images based on increasingly complex prompts, allowing for more nuanced and detailed artworks.
- Address ethical considerations and intellectual property concerns as the lines between human creativity and algorithmic generation blur.
- Foster collaboration between artists and AI, where artificial intelligence serves as a tool to expand creative possibilities and not as a replacement for human ingenuity.
The technical progression enables AI to create images that mimic existing art styles and innovate new forms, challenging the conventional boundaries of artistic expression. Neural networks like DALL·E 2 and Jasper Art illustrate the potential for AIs to produce unique and imaginative artworks. However, as we leverage AI art generators, it is crucial to navigate the ethical implications and intellectual property issues that arise when using AI art, ensuring a fair and respectful integration of technology in the creative domain.
Ethical Implications of AI-Produced Art
As the prevalence of AI in art creation surges, it is imperative to scrutinize the ethical implications accompanying this technology’s emergence. This includes considerations regarding copyright, originality, and the potential perpetuation of biases.
Integrating artificial intelligence into the realm of creativity prompts a reevaluation of what constitutes the authorship and ownership of works of art. The realism of the generated pieces by AI art generators challenges traditional notions of authenticity, raising questions about the preservation of human creativity in the age of mechanization.
The use of AI to create art is full of legal considerations; it necessitates clear guidelines to determine the rights associated with AI-produced works. Moreover, creating art through AI raises whether the algorithm or the human operator should be credited or if a new category of shared authorship should be recognized.
Furthermore, as AI systems are trained on existing data, there is a risk of replicating and amplifying societal biases within the art they produce. Therefore, The ethical responsibility extends to the artists, developers, and organizations deploying these technologies.
Ethical use of AI art requires transparency, accountability, and an ongoing dialogue to address the complex interplay between artificial intelligence and human expression.
FAQs
What role do neural networks play in art creation?
Neural networks in art creation serve as computational models that can generate, modify, or enhance artistic content. They learn patterns and styles from existing artworks and use that knowledge to produce new and unique pieces.
Which neural networks are considered top choices for art creation?
Some top neural networks for art creation include Generative Adversarial Networks (GANs), Variational Autoencoders (VAEs), and Neural Style Transfer (NST). Each has its approach to generating or transforming visual content.
How do Generative Adversarial Networks (GANs) contribute to art creation?
GANs consist of a generator and a discriminator that work in opposition. The generator creates new art, and the discriminator evaluates it. This adversarial process results in the generation of realistic and diverse artworks.
In what ways are Variational Autoencoders (VAEs) used in art creation?
VAEs are used in art creation to learn latent representations of input data, allowing for generating new and diverse artworks. They are often employed to explore the latent space of artistic styles.
What is Neural Style Transfer (NST), and how does it impact art creation?
Neural Style Transfer is an algorithm that applies the artistic style of one image to the content of another. It transforms art by blending the style of well-known artworks or chosen styles with existing digital images.