AI Art Generation: Top ML Models
The advent of artificial intelligence in art generation has given rise to an intriguing intersection of technology and creativity. Among the vanguard of this innovative fusion are several prominent machine-learning models that have redefined the boundaries of automated artistic expression.
Generative Adversarial Networks (GANs), Variational Autoencoders (VAEs), and Transformer models, such as OpenAI’s DALL-E, stand at the forefront of this digital renaissance, each with its unique approach to synthesizing imagery that resonates with both aesthetic allure and technical sophistication.
As we examine these models, we must consider their artistic capabilities and the underlying algorithms that enable their function, the quality and diversity of output they produce, and the ethical implications they carry in the broader scope of creative authorship.
The ensuing discourse aims to unpack these dimensions, setting the stage for a nuanced exploration of how these technologies shape art’s future.
Key Takeaways
- GANs and VAEs are key machine learning models for AI art generation. GANs focus on creating realistic and detailed artworks, and VAEs generate new variations and explore artistic expression.
- RNNs revolutionize sequential data processing in AI art generation, allowing for generating coherent sequences such as image generation from text prompts or music composition.
- Transformer models capture long-range dependencies in data sequences and transform the landscape of AI art generation by enabling real-time generation and producing highly original and intricate digital art.
- CNNs are crucial in generating unique and precise images, extracting intricate details from images, and optimizing digital art creation. They have revolutionized AI-driven artistry and redefined the boundaries of image-based machine learning.
Generative Adversarial Networks (GANs)
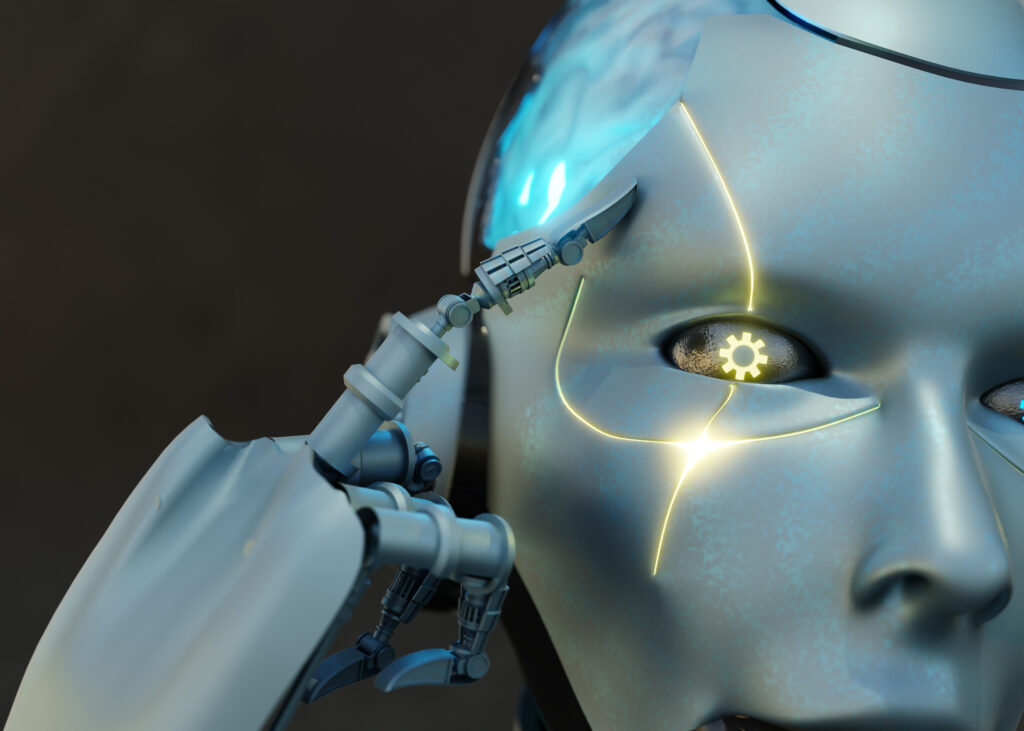
Generative Adversarial Networks (GANs) have revolutionized the field of AI art generation by leveraging the dynamic interplay between their dual-network architecture to synthesize visually compelling and authentic images. Operating at the forefront of generative AI, GANs employ a pair of neural network models to generate new images that are often indistinguishable from original artworks.
The generative model, known as the generator, crafts data instances, which are then scrutinized by the discriminative model, aptly termed the discriminator. This iterative adversarial process hones the generator’s ability to create realistic images with astonishing detail and complexity.
The discriminator’s evaluative role is pivotal, guiding the generator to produce more convincing outputs. As a result, GANs have become instrumental generative AI tools in the domain of AI to create art. The models to generate such art are trained on large datasets of existing artworks, enabling the generator to assimilate diverse artistic styles and elements. This training culminates in the generator’s capacity to produce unique and intricate art pieces.
Implementing GANs using AI Art generators has significantly advanced the technology’s ability to emulate human creativity. The refined images produced by such systems exemplify the AI art generation’s potential to mimic and innovate in artistic expression.
Variational Autoencoders (VAEs)
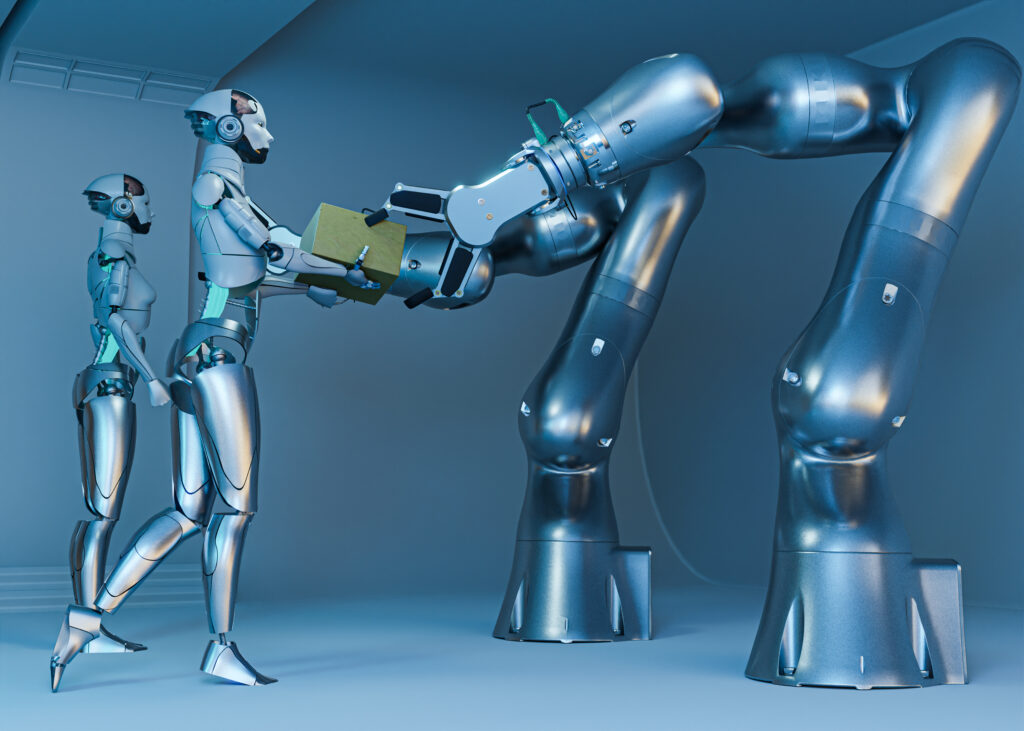
Building upon the innovation of Generative Adversarial Networks in the realm of AI art, Variational Autoencoders introduces a different approach, focusing on the encoding and decoding of data to facilitate the generation of new artistic expressions. VAEs stand out for their robustness and versatility in art generator use by employing a probabilistic twist to the autoencoder architecture. These models excel in creating a diverse range of outputs by learning to reconstruct inputs with variations, making them ideal for using AI to generate unique images.
VAEs contribute to the realm of AI art in several critical ways:
- They create a structured latent space, which art generators use to produce new variations with smooth interpolations.
- Unlike deterministic models, VAEs generate unique images by sampling from a probability distribution, enhancing creativity.
- Their inherent ability to learn complex distributions allows image generators to produce images based on text descriptors, contributing to the expanding domain of AI-driven art.
Recurrent Neural Networks (RNNs)
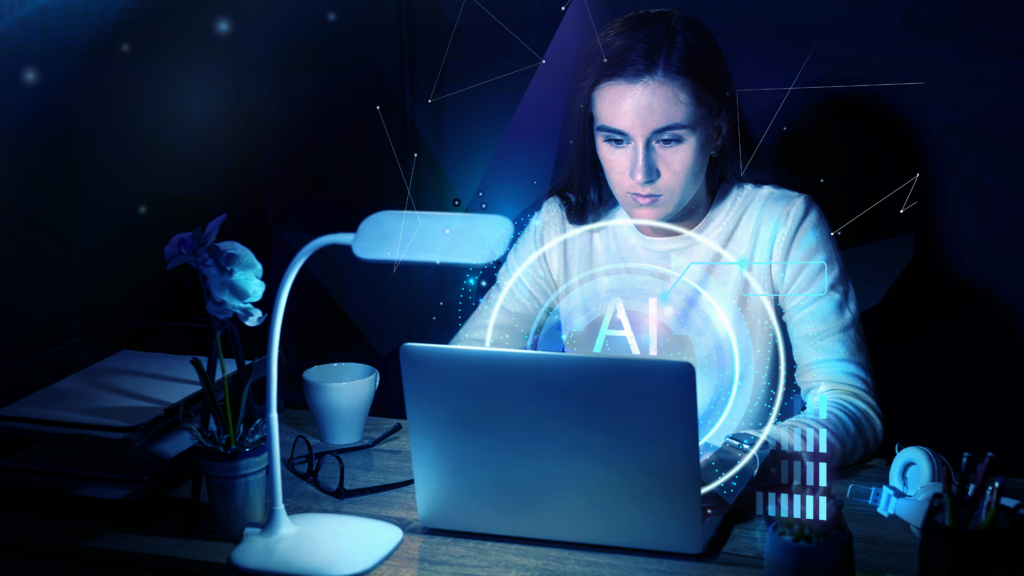
Recurrent Neural Networks (RNNs) revolutionize sequential data processing by leveraging their unique capacity to maintain a form of memory across input sequences. In the context of Artificial Intelligence (AI), particularly in AI art generation, RNNs offer significant advantages. Their inherent structure is adept at generating new, coherent sequences, which can be applied to creating images from text prompts or music composition.
RNNs’ ability to process variable-length input sequences makes them well-suited for tasks such as generating images where continuity and context are essential. When developing AI art, RNNs can be trained to generate multiple images that follow a narrative or thematic sequence, creating unique images that tell a story.
Enhanced architectures like LSTM and GRU networks notably address the limitations of the vanishing gradient problem in traditional RNNs. These powerful AI models retain the ability to capture long-term dependencies, which is critical in generating coherent and sophisticated visual art, such as AI-generated portraits or intricate patterns.
Despite these advancements, RNNs must still be challenged when generating high-resolution imagery. Consequently, they are often employed with other neural network models, like Convolutional Neural Networks (CNNs), to amplify their capabilities in art generation, allowing them to create more complex and detailed visual content.
Transformer Models
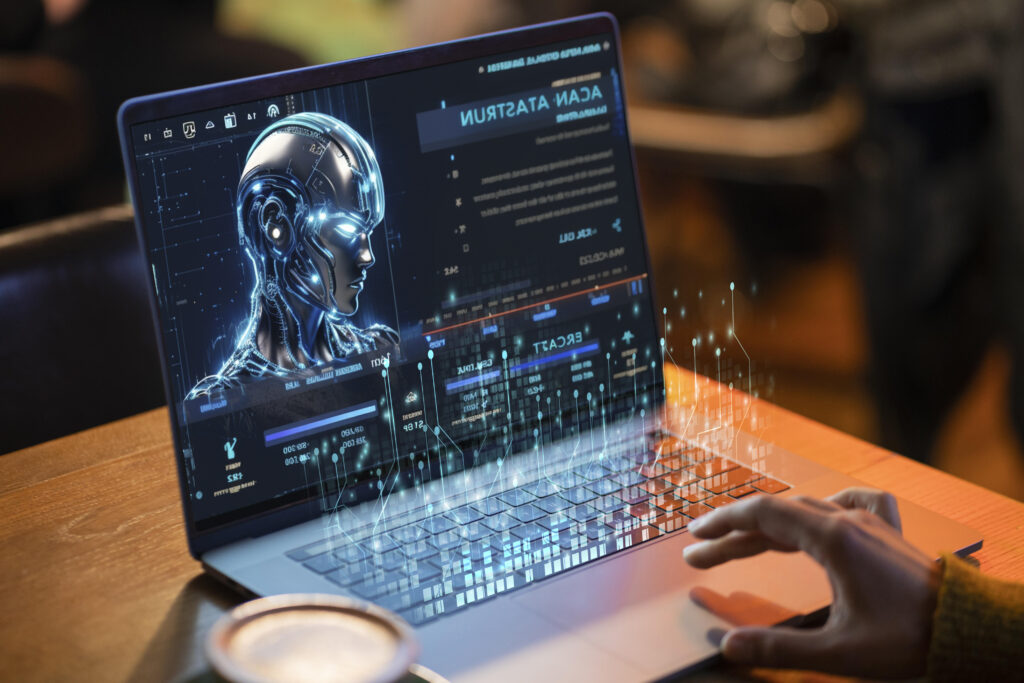
While RNNs have carved a niche in sequential data processing, transformer models have emerged as a powerful alternative, particularly suited for capturing long-range dependencies in data sequences. The advent of transformer models has been a game-changer in machine learning, especially in the generation of digital art.
Transformer models bring several advantages to AI art generators:
- They efficiently process entire data sequences in parallel, shortening training times and enabling real-time generation.
- The self-attention mechanism allows the model to focus on different parts of the input sequence, which is critical for understanding the context and nuances within text prompts.
- Their highly adaptable architecture makes them ideal for the iterative development and fine-tuning required in AI-driven creative processes.
In the context of AI art generation, transformer models like OpenAI’s DALLE-2 have demonstrated remarkable capabilities. By interpreting text prompts, these generators can produce highly original and intricate digital art, reflecting the subtlest intentions of the user’s input. Midjourney, another such model, leverages machine learning advancements to generate compelling imagery that pushes the boundaries of AI creativity.
As transformer models continue to evolve, they are setting new benchmarks for what machines can create, transforming the landscape of AI art generation.
Convolutional Neural Networks (CNNs)
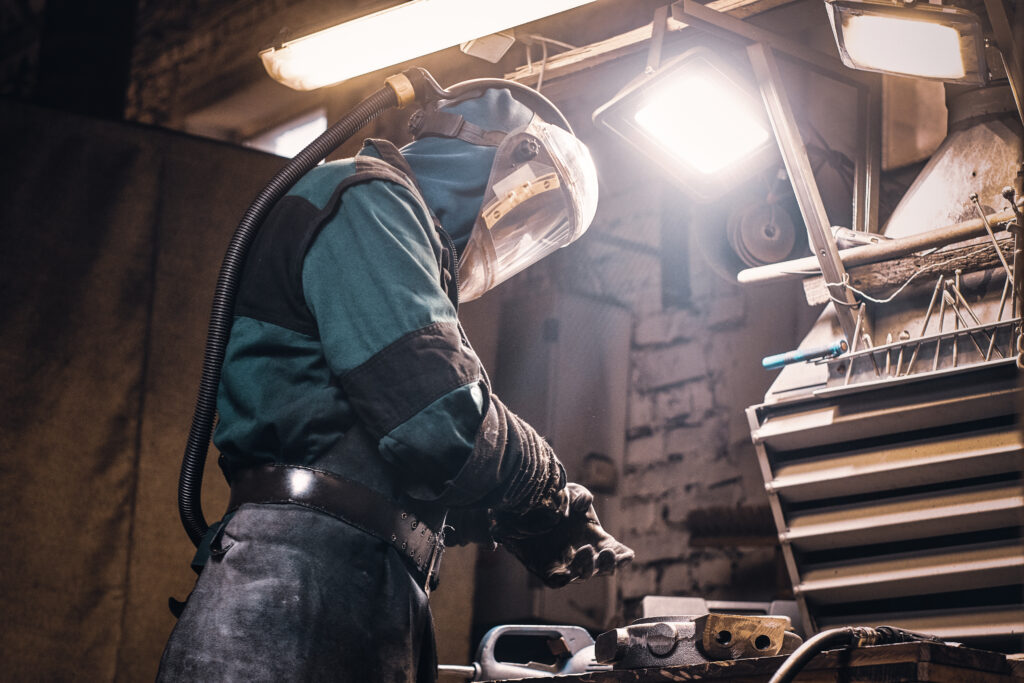
Convolutional Neural Networks (CNNs) stand at the forefront of image analysis, utilizing layered architectures that mimic biological visual systems to extract and learn complex patterns from visual data. Characterized by their capacity to automatically learn spatial hierarchies of features, CNNs optimize the process of creating digital art by enabling the efficient manipulation of images. Their inherent structure, comprising convolutional, pooling, and fully connected layers, empowers them to discern intricate patterns and features, a vital attribute for tasks like photo editing, where precision is paramount.
These networks are adept at applying various art styles, offering artists the tools to edit and create unique images based on their vision. The efficiency of CNNs in handling voluminous and complex image datasets renders them indispensable in visual art generation.
FeatureSignificance in AI Art Generation | |
---|---|
Pattern Recognition | Extracts intricate details from images. |
Hierarchical Learning | Learns and applies diverse art styles. |
Adaptability | Adapts to create unique images with precision. |
Employing a systematic approach, CNNs have redefined the boundaries of image-based machine learning, playing a crucial role in the evolution of AI-driven artistry.
FAQs
What role do machine learning models play in AI art generation?
Machine learning models, particularly generative models like GANs (Generative Adversarial Networks) and VAEs (Variational Autoencoders), are used in AI art generation to learn patterns from data and generate new, creative artworks.
Which machine learning models are famous for AI art generation?
Some popular machine learning models for AI art generation include GANs, VAEs, StyleGAN, Neural Style Transfer (NST), and deep learning models like convolutional neural networks (CNNs) and recurrent neural networks (RNNs).
How do GANs contribute to AI art generation?
GANs consist of a generator and a discriminator that compete against each other during training. The generator creates art, and the discriminator evaluates it. The adversarial process results in the generation of realistic and diverse artworks.
What is the significance of Variational Autoencoders (VAEs) in AI art generation?
VAEs encode and decode images, capturing latent representations of data. In AI art generation, VAEs are valuable for creating diverse and novel artworks by manipulating latent features.
Can StyleGAN be considered a prominent machine learning model for AI art?
StyleGAN is a prominent machine-learning model known for generating high-quality and realistic images with fine control over style elements. It has been widely used for creating visually appealing AI-generated art.