AI Art Excellence: Top 5 Advanced GAN Algorithms
In recent years, Generative Adversarial Networks (GANs) have significantly advanced, unveiling a new era in AI art generation that blends technological sophistication with creative expression.
Among the plethora of GAN algorithms, a few stand out for their groundbreaking capabilities, altering the landscape of digital artistry. StyleGAN, for instance, has carved a niche by generating hyper-realistic images, pushing the boundaries of quality and resolution in AI-generated artwork.
Meanwhile, BigGAN’s prowess in scaling up imagery without losing detail has earned it a spot among the top contenders. CycleGAN’s unique approach to unpaired image-to-image translation offers artists a new level of transformational flexibility. In contrast, GauGAN’s interactive landscapes have introduced unprecedented user engagement in art creation.
Lastly, VQ-VAE-2’s ability to synthesize high-resolution images heralds a future where AI can mirror the intricacies of human artistry.
As we unveil the salient features and applications of these top-tier GANs, it becomes evident that each algorithm harbors its strengths and potential implications for the future of AI-generated art, raising questions about the evolving relationship between artificial intelligence and human creativity.
Key Takeaways
- StyleGAN and BigGAN are pioneering GAN algorithms that push the boundaries of AI-driven creativity and generate highly detailed and authentic images.
- CycleGAN enables the transformation of visual elements between domains without paired training examples, allowing for the creation of digital art in many unique styles.
- GauGAN empowers users to transform rudimentary sketches into realistic landscape images through interactive design and converts text-to-image AI prompts into unique works of art.
- VQ-VAE-2 synthesizes high-resolution images with remarkable detail and clarity, making it an essential tool for artists and developers requiring precision in image generation.
StyleGAN: Pioneering Quality
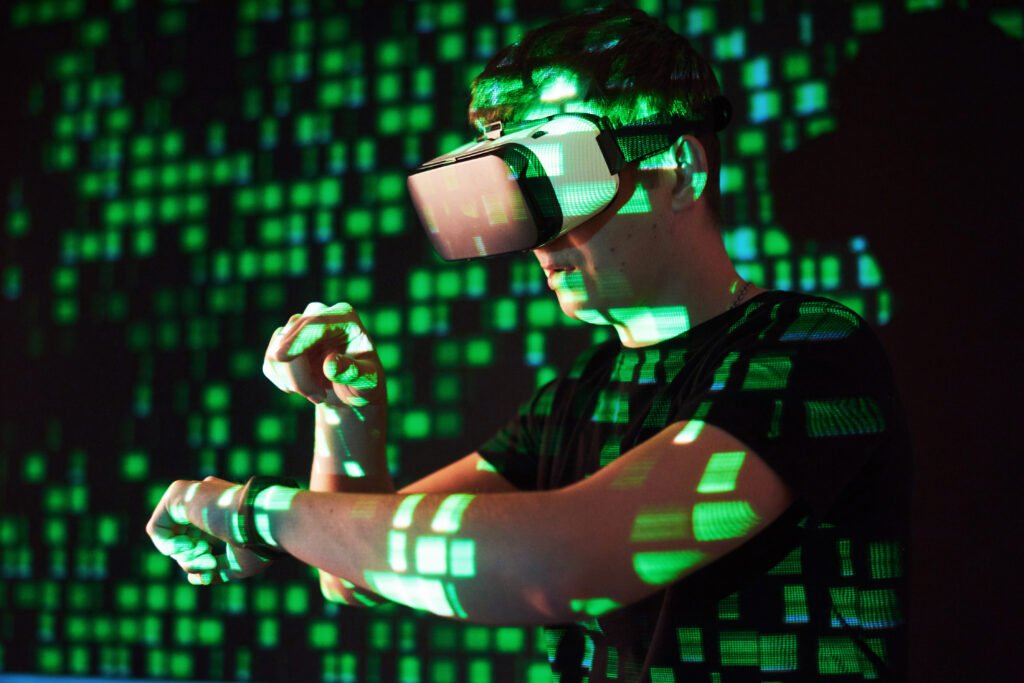
How does StyleGAN redefine the landscape of AI-generated art by producing unprecedented quality and detailed images?
As a generative AI algorithm specializing in art generation, StyleGAN represents a significant leap forward in the capabilities of AI art generators. Developed on artificial intelligence (AI) principles, this advanced model is engineered to generate unique, highly detailed images that push the boundaries of what is possible in AI-driven creativity. StyleGAN’s innovative architecture enables it to produce outstanding artwork with an authenticity that closely mirrors real-world objects and scenes.
The versatility of StyleGAN lies in its extensive customization options, which empower users to exert fine control over various aspects of the image generation process. This flexibility facilitates the creation of AI-generated portraits and landscapes in many artistic styles, catering to a wide array of aesthetic preferences. StyleGAN’s proficiency in rendering intricate textures and nuances contributes to its recognition as a pioneering force in the generative AI space.
These qualities make StyleGAN an indispensable tool for artists and technologists exploring the intersection of technology and art through the lens of AI.
BigGAN: Scaling Up Imagery
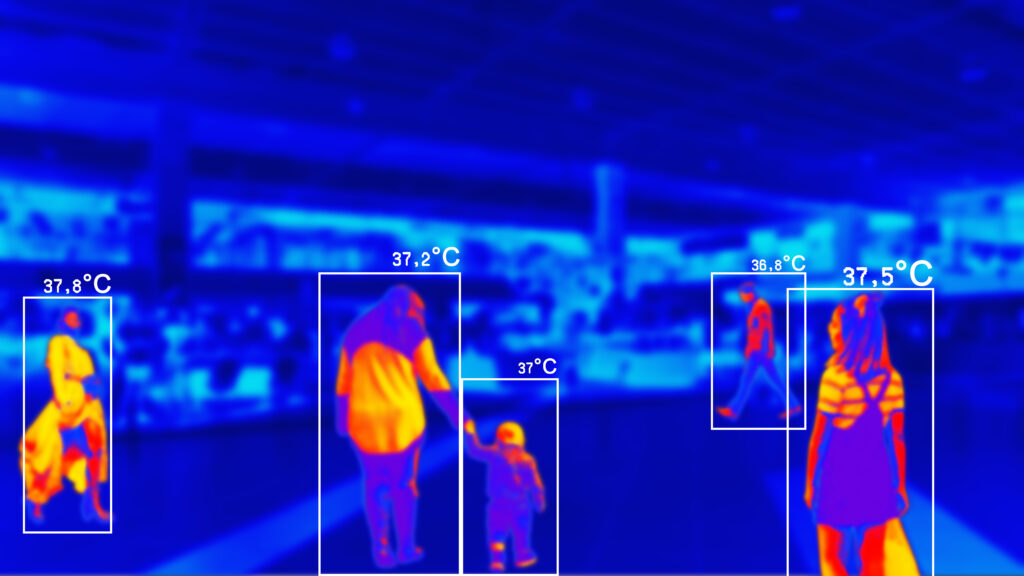
BigGAN distinguishes itself as an advanced generative adversarial network that excels in producing large-scale, high-resolution imagery with remarkable detail and diversity, thereby setting a new benchmark for image quality in AI art generation. As a pivotal innovation among AI art generators, BigGAN is designed to generate unique images that push the boundaries of what is visually possible with AI algorithms. This remarkable ability to scale up imagery without losing quality enables it to produce unique and intricate visuals across various categories.
- High-Resolution Output: BigGAN leverages increased computational power to generate images with unprecedented resolution, making it a preeminent choice for applications requiring detailed visuals.
- Diversity and Realism: It exhibits a profound capacity to produce various images based on different inputs while maintaining realism even at large scales.
- Professional Use: The advanced GAN algorithm is particularly suited for professional-grade AI art generation, providing artists and creators with a robust tool to envision and materialize high-fidelity art pieces.
CycleGAN: Unpaired Transformations
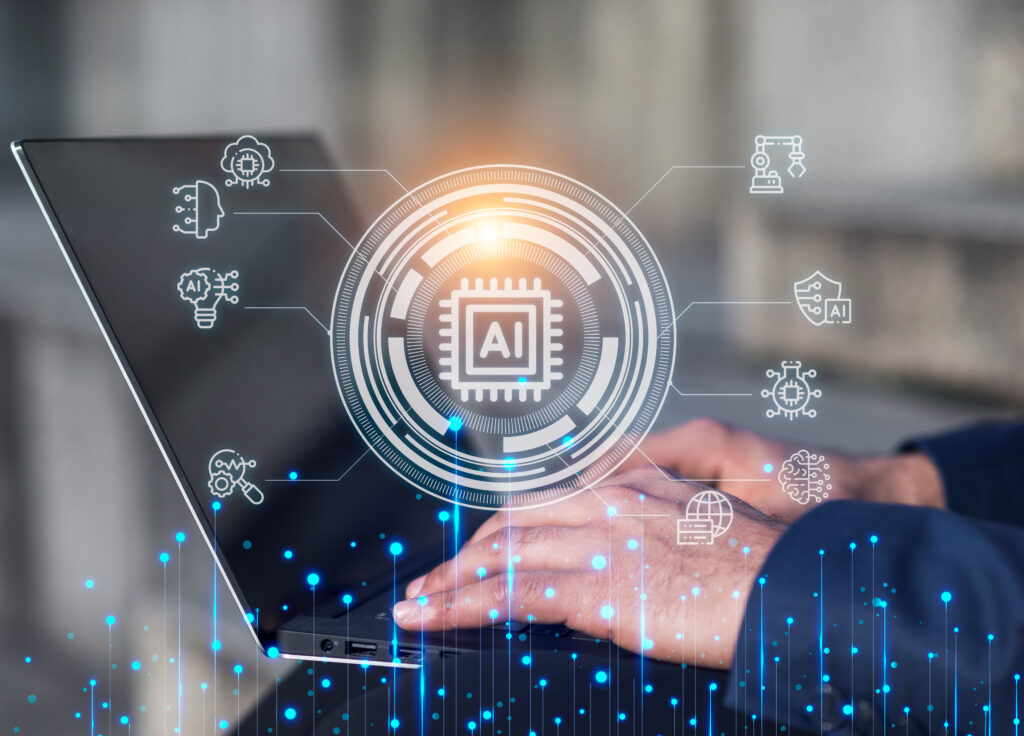
CycleGAN revolutionizes the field of image translation by enabling the transformation of visual elements from one domain to another without using paired training examples. This advanced GAN framework is distinguished by its capacity to learn and apply unpaired transformations, facilitating digital art creation in many unique styles.
Leveraging the concept of cycle consistency, CycleGAN effectively ensures that an image can be translated from one domain to a target domain and then back again, preserving the original content while adapting the style.
The AI-driven art generators that employ CycleGAN are adept at generating new, authentic artwork by harnessing these unpaired transformations. The algorithm’s strength lies in its ability to absorb and reinterpret diverse art styles, thus generating novel images that maintain a high degree of aesthetic and structural coherence.
The unpaired nature of the transformation process that CycleGAN undertakes eliminates the need for meticulously matched image pairs, which often need to be more cohesive and practical to curate.
GauGAN: Interactive Landscapes
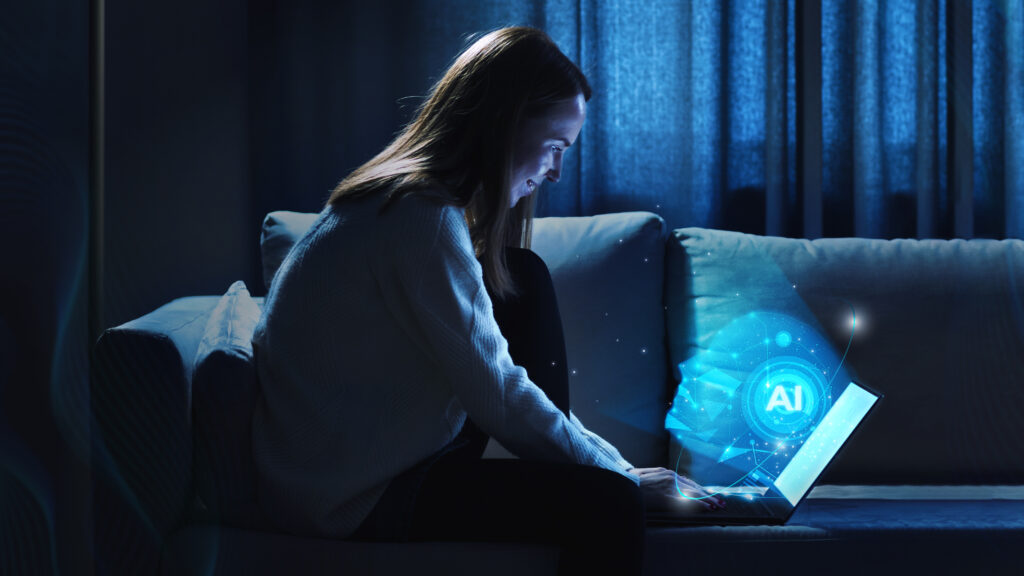
GauGAN, a state-of-the-art AI art generator, transforms rudimentary sketches into strikingly realistic landscape images by applying advanced generative adversarial networks. This innovative tool has revolutionized the creation of interactive landscapes, allowing users to generate natural scenes from simple inputs. By interpreting elemental brushstrokes, GauGAN enables the composition of detailed environments that resonate with the complexity and beauty of natural landscapes.
Critical aspects of GauGAN include:
- Interactive Design: Empowers users to craft landscapes intuitively, transforming basic sketches into detailed art.
- Advanced AI: Utilizes GANs to process and render images that closely mimic real-world textures and lighting.
- Versatility: Can interpret even abstract concepts and translate them into visually coherent scenes.
As a pinnacle of AI art generators, GauGAN stands out for its capacity to convert text-to-image AI prompts into unique works of art. Artists and non-artists alike can use tools provided by GauGAN to create immersive and intricate landscapes without the need for extensive artistic training. The sophistication of AI generation within GauGAN lies in its ability to understand and replicate the nuances of natural elements, thereby enabling the creation of art that was once confined to the imagination.
VQ-VAE-2: High-Resolution Synthesis
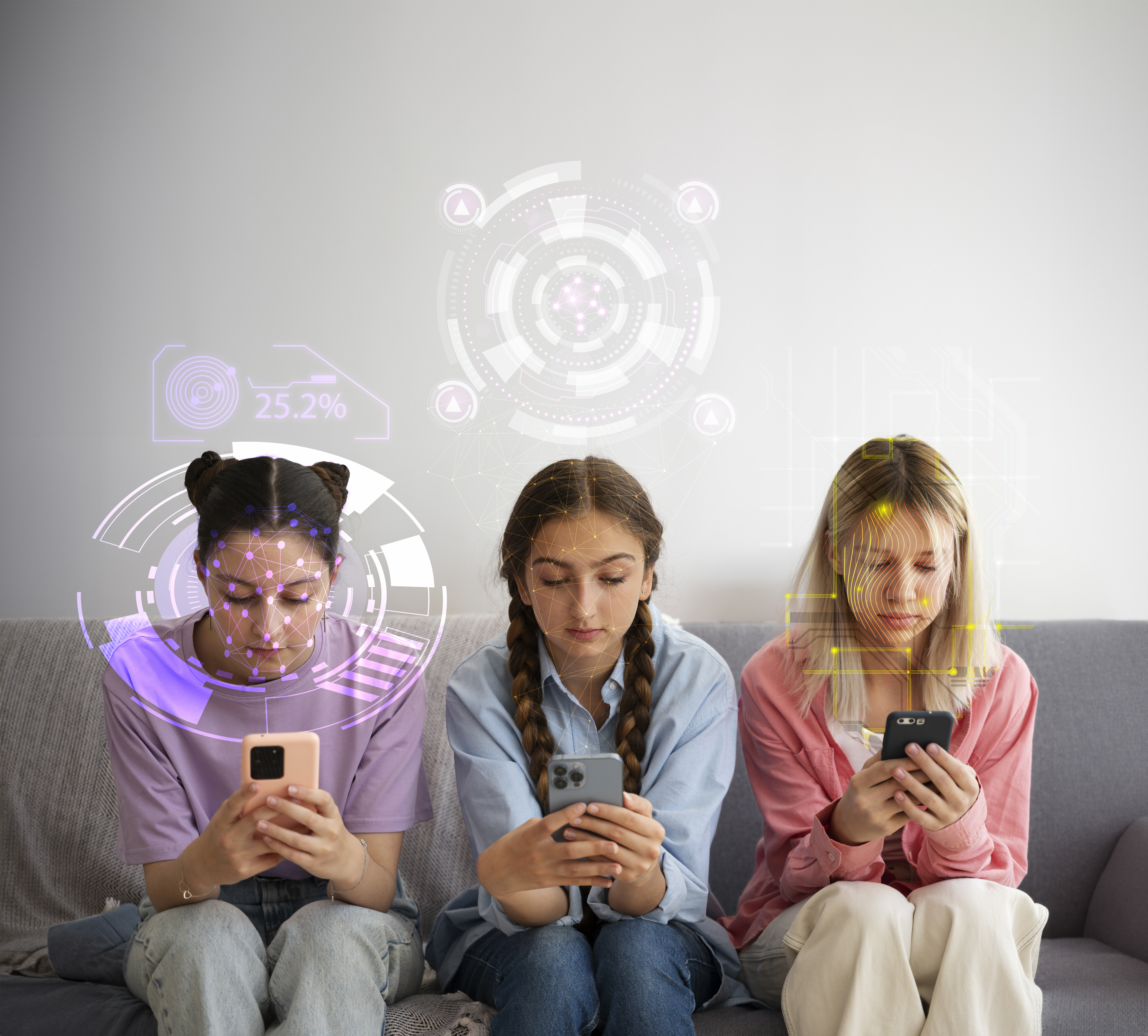
Building upon the foundations of generative art, VQ-VAE-2 emerges as a sophisticated algorithm designed to synthesize high-resolution images with remarkable detail and clarity. This advanced model leverages the principles of vector quantization combined with variational autoencoders to generate visual art that is both intricate and photorealistic.
VQ-VAE-2’s proficiency lies in its ability to produce unique images that exhibit high fidelity, catering to the demands of high-resolution synthesis in AI art generators.
The algorithm’s architecture is fine-tuned to generate complex and elaborate artwork, positioning it as an essential tool for artists and developers who require tools to generate images per prompt with precision. Its strength in creating photorealistic textures and patterns positions VQ-VAE-2 as an invaluable asset within the arsenal of AI art generators.
FAQs
What role does ProGAN play in advancing the quality of AI-generated art?
ProGAN, or Progressive GAN, focuses on progressively increasing the resolution of generated images during training. This results in high-quality and detailed AI art, making it suitable for applications requiring fine details.
Can these advanced GAN algorithms be used for various forms of AI art, not just images?
While these GAN algorithms are commonly used for image generation, they can also be adapted for other forms of AI art, such as video synthesis, style transfer in videos, and more. The principles behind GANs can be applied to various creative domains.
What considerations should artists keep in mind when using advanced GAN algorithms?
When using advanced GAN algorithms, artists should consider the computational requirements, training data, and ethical implications. It’s essential to experiment, fine-tune parameters, and stay updated on developments in GAN research.
Are there resources or tutorials available for artists interested in using these advanced GAN algorithms?
There are various online resources, tutorials, and community forums where artists can learn and share their experiences with advanced GAN algorithms. Platforms like GitHub and AI research conference proceedings are valuable sources of information.
How can artists overcome challenges when working with advanced GAN algorithms?
Challenges can be overcome by experimenting with pre-trained models, understanding the nuances of training parameters, seeking community support, and staying informed about best practices in GAN-based AI art.