Crafting AI Art: GAN Technology
In recent years, the intersection of artificial intelligence and the creative arts has given rise to a new paradigm in which Generative Adversarial Networks, or GANs, have taken center stage. This sophisticated form of AI enables the generation of visual content often indistinguishable from that created by human hands, challenging our traditional notions of artistry and creativity.
As we consider the intricate dance between the generator, which produces the art, and the discriminator, which critiques it, we cannot help but ponder the broader implications of this technology. From the potential to democratize art creation to the profound questions it raises about originality and authorship, GAN technology is not just reshaping the artistic landscape but also propelling us into an era of unprecedented digital expression.
As we turn our gaze to this evolving domain, one must contemplate the intricate balance between the augmentation of human creativity and the rise of autonomous artistry. This subject warrants further exploration and discussion.
Key Takeaways
- GANs, or Generative Adversarial Networks, are AI algorithms that consist of a Generator and a Discriminator.
- GANs have significantly impacted art, blurring the lines between human and machine creativity.
- GAN-generated art has challenged traditional aesthetic values and opened up new possibilities for digital art.
- Using GANs in art raises ethical questions and requires responsible practices to navigate issues of ownership and authorship.
Understanding GANs
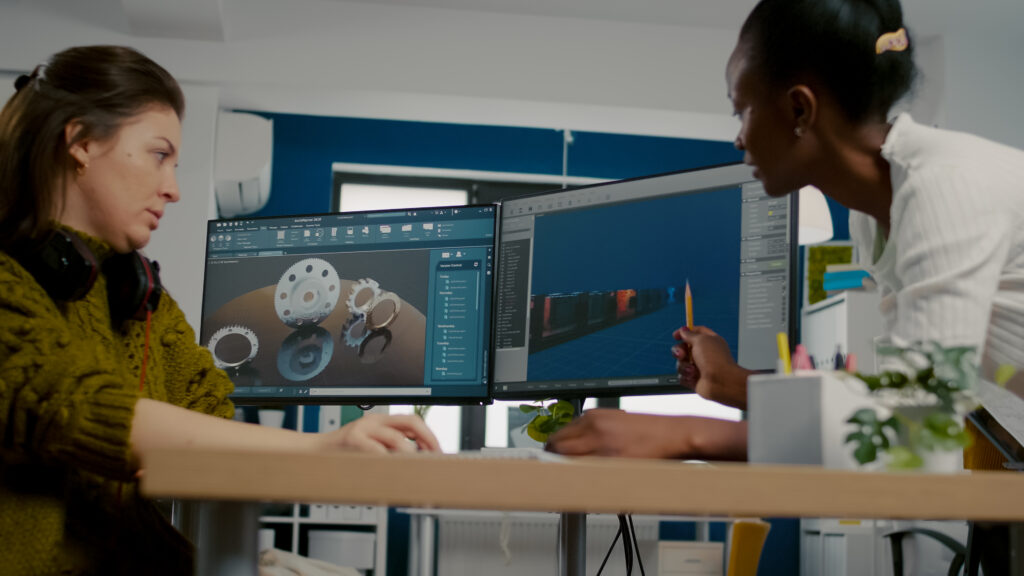
Generative Adversarial Networks (GANs) are a class of artificial intelligence algorithms that involve a dualistic interplay between two neural networks, the Generator, and the Discriminator, to produce new, synthetic data instances indistinguishable from actual examples.
The Generator’s role is to fabricate data, such as images, that simulate the distribution of genuine datasets. Conversely, the Discriminator evaluates the authenticity of the data, discerning between the Generator’s output and actual data.
The two networks engage in a continuous contest: the Generator strives to enhance its capacity to produce convincing data, while the Discriminator becomes more adept at detecting forgeries.
This adversarial process is analogous to a game of deception and detection, where each component refines its strategy through iterative training.
Since their inception in 2014, GANs have rapidly advanced, securing a pivotal role across various sectors. GANs empower the synthesis of intricate and realistic textures and assets in art and gaming.
Within healthcare, they assist in generating medical imagery for research and training. However, training GANs poses substantial technical challenges, and the generation of synthetic media raises ethical questions regarding authenticity and misuse.
Despite these challenges, GANs continue to be a driving force for innovation, facilitating creative expression and expanding the frontiers of artificial intelligence.
GAN Architecture Explained
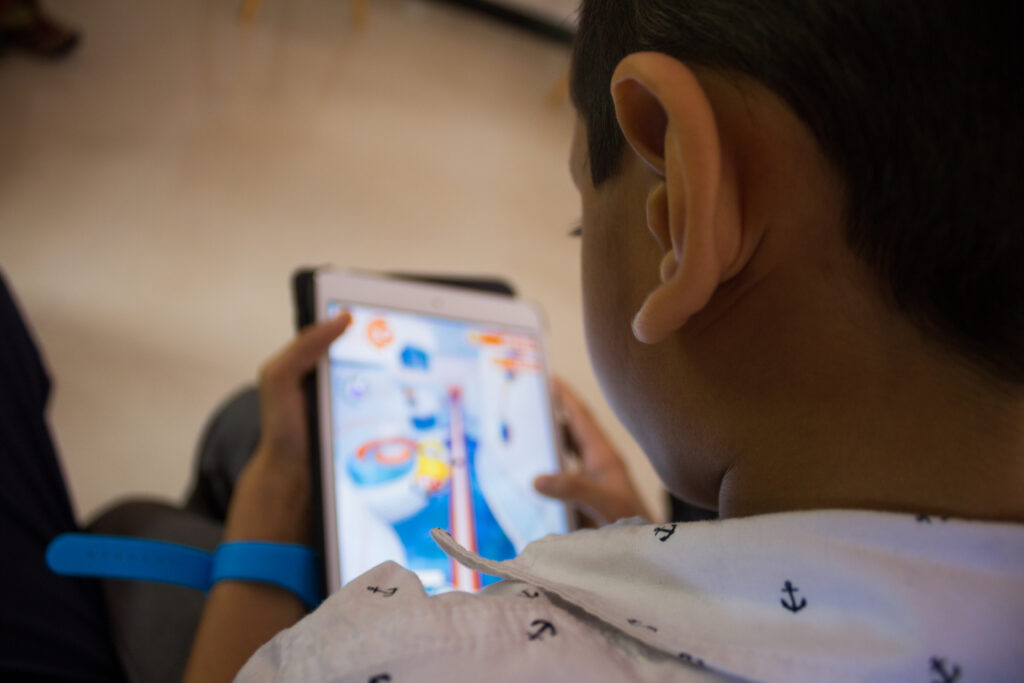
How do the interconnected networks within a GAN function to create convincing synthetic media?
The GAN model comprises two distinct yet collaborative neural networks: the Generator and the Discriminator. The architecture is designed to engage these networks in a continuous strategic game. While the Generator is tasked with producing artificial content, the Discriminator evaluates the content against actual data. This adversarial process is iterated until the Generator’s output is indistinguishable from authentic data, effectively fooling the Discriminator.
The intricacies of the GAN architecture can be summarized as follows:
- Generator Network: It begins with a random noise input and transforms it through a series of layers to create data that mimics the desired distribution. Its success is measured by its ability to produce images that the Discriminator cannot reliably label as fake.
- Discriminator Network: Operating as a binary classifier, it scrutinizes images to determine the likelihood that they are either genuine or artificial. The Discriminator provides critical feedback to the Generator, which informs subsequent refinements in the generated images.
- Adversarial Training: The crux of a GAN model lies in the negative training process where the Generator and Discriminator improve through competition. The Generator progressively enhances its capability to create realistic images while the Discriminator becomes more adept at detection, leading to a sophisticated equilibrium.
The GAN architecture’s potency is in its elegant adversarial dynamics, which drive the networks toward creating high-fidelity synthetic media.
Training GANs for Art
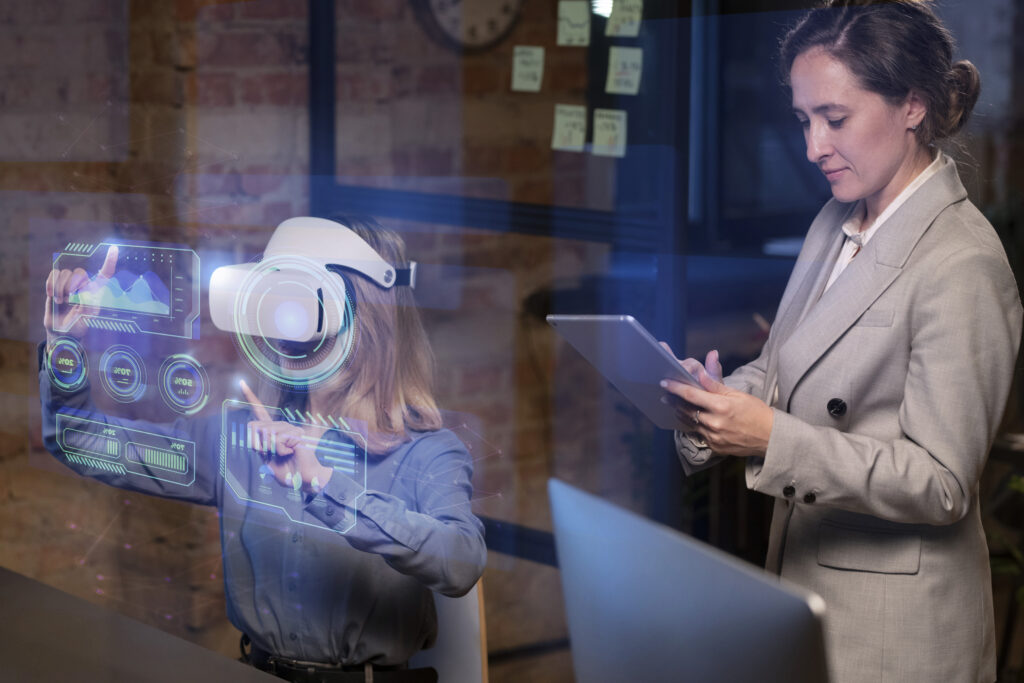
Training Generative Adversarial Networks (GANs) for art requires a nuanced approach that includes curating extensive art datasets and deploying substantial computational power to guide the learning process effectively. These AI models are composed of two parts: the Generator, tasked with creating artwork, and the Discriminator, which learns to distinguish between genuine and synthetically produced art. These models’ success hinges on the input data’s breadth and quality. The Generator is exposed to various artistic styles by ingesting vast datasets, enabling it to produce diverse and complex outputs.
Moreover, the computational demands of training GANs are significant. They necessitate powerful GPUs and often distributed computing resources to efficiently iterate through the adversarial learning cycles. Each iteration refines the model’s capabilities, gradually improving the authenticity of the generated art.
Ethical considerations are also at the forefront of training GANs for art. Addressing the potential for misuse, such as creating deepfake content, is imperative while fostering transparency and accountability in the AI’s creative process. Through careful training and ethical oversight, GANs can lead to innovative art projects and contribute to expanding digital art frontiers.
Pioneering GAN Artworks
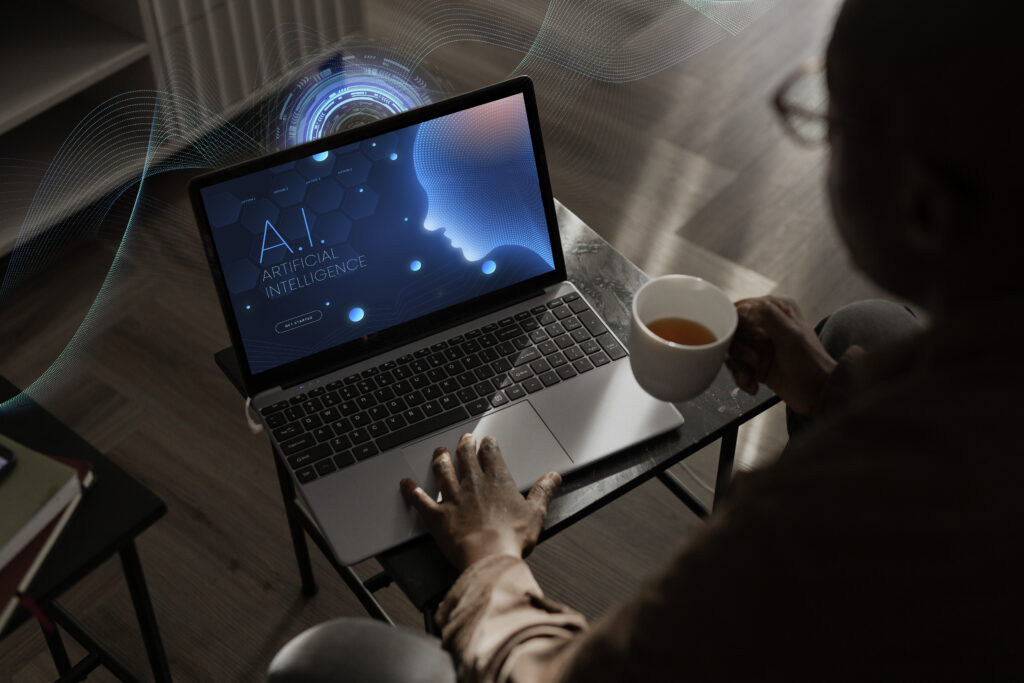
The inception of GAN technology has given rise to a new genre of art. This genre is characterized by early masterpieces that blurred the lines between human and machine creativity. Breakthrough artists produced these pioneering works. They challenged traditional aesthetic values and charted the evolution of a distinct GAN-influenced style.
As we examine these seminal artworks, we must consider the technical innovation they represent and their broader impact on the art world’s visual lexicon.
Early GAN Masterpieces
Pioneering GAN artworks, often considered masterpieces, have demonstrated the early capabilities of Generative Adversarial Networks within digital art. These early GAN masterpieces laid the groundwork for subsequent explorations in AI-driven creativity and marked a significant milestone in the intersection of technology and visual arts.
The evolution and influence these groundbreaking works had on the art community are underscored by several key aspects:
- Innovation: Early GAN masterpieces introduced novel aesthetic dimensions, challenging traditional perceptions of creativity and originality.
- Inspiration: They sparked a wave of experimentation among artists and technologists eager to explore the untapped potential of AI in art.
- Impact: The artworks catalyzed discussions on the role of AI in the future of artistic expression, altering the trajectory of digital art.
Breakthrough Artists and Works
Breakthrough artists utilizing GAN technology have redefined the landscape of digital art with innovative creations ranging from stylistic emulations of classic masters to the conception of unprecedented visual languages.
These avant-garde figures have propelled GAN artworks into the limelight of art exhibitions, where the fusion of algorithmic complexity and aesthetic inquiry captivates diverse audiences. Their works, exemplars of AI-generated art, have not only graced research publications but also spurred a multitude of creative projects.
GAN’s Aesthetic Evolution
Amidst the rapid advancement of generative adversarial networks (GANs), a diverse array of pioneering artworks has emerged, marking a significant aesthetic evolution in digital creativity. These developments reflect the following facets:
- Innovative Approaches to Art Generation: GANs have redefined the processes of creating art, enabling artists to explore complex, multidimensional aesthetic spaces that were previously inaccessible.
- Challenges in Training: The intricate training of GANs, with potential issues like mode collapse, necessitates substantial datasets and computational power, pushing the technical boundaries of art generation.
- Ethical and Aesthetic Implications: As GANs disrupt traditional art paradigms, they raise ethical questions and alter aesthetic judgments, prompting a reevaluation of the creator’s role and the nature of creativity.
Commercial GAN Applications
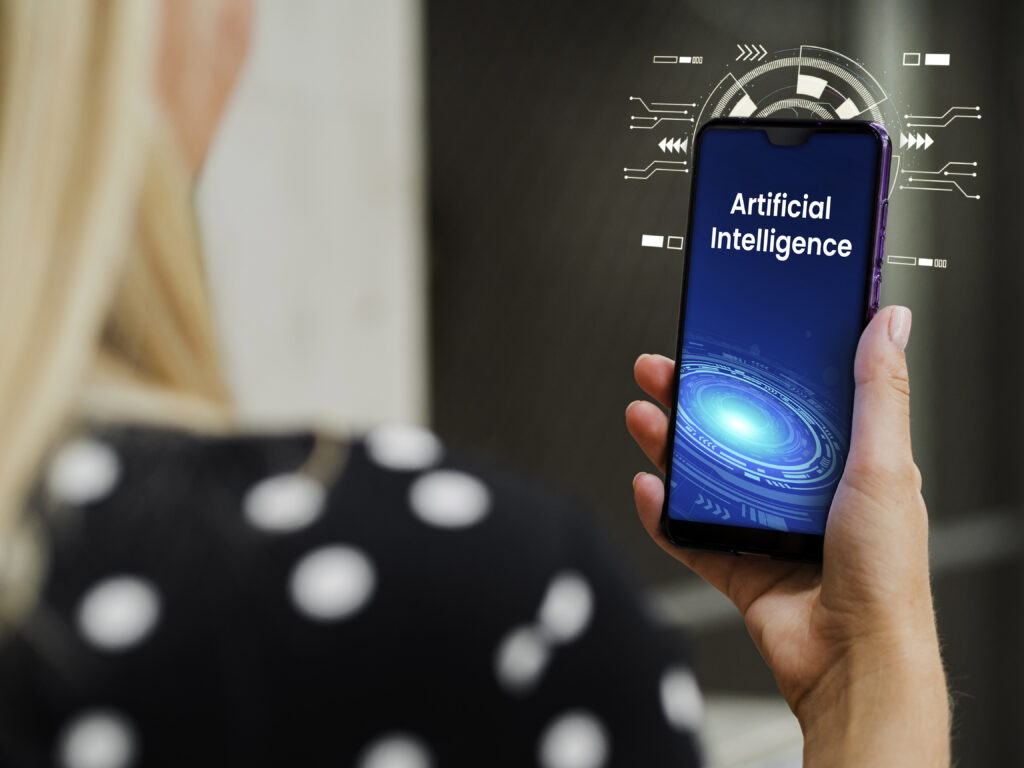
Generative Adversarial Networks (GANs) have been increasingly adopted in commercial sectors, revolutionizing the creation and modification of digital content across various industries. GANs have introduced a paradigm shift in art and design by generating innovative and unconventional perspectives that challenge traditional creative processes. This has enabled artists and designers to explore new frontiers of creativity, yielding previously inconceivable outputs.
GANs enhance immersive experiences in the gaming industry by constructing ultra-realistic environments and characters, elevating games‘ visual fidelity and engagement. The technology’s ability to generate high-quality synthetic data has proven invaluable in creating detailed virtual worlds without extensive real-world data capture.
The healthcare sector has been another significant beneficiary of GAN applications, particularly in generating synthetic medical imagery. By producing accurate and diverse medical images, GANs aid in training diagnostic algorithms, improving their robustness and reliability in clinical settings.
However, the advent of deep fakes—a direct offshoot of GAN capabilities—has sparked ethical debates. The potential for misuse in creating convincingly altered videos or images poses risks to information integrity, necessitating a cautious approach to deploying GANs in commercial applications.
Artistic Implications of GANs
Exploring the intersection of technology and creativity, Generative Adversarial Networks (GANs) have ushered in a new era in art and design, fundamentally altering the landscape of artistic expression and generation. The creative implications of GANs are profound, as they redefine the creation and consumption of art through advanced computational processes.
GANs facilitate the emergence of captivating and intellectually challenging artworks, pushing the boundaries of traditional aesthetics and conceptual frameworks.
The following list highlights three significant artistic implications of GANs:
- Expansion of Creative Boundaries: GANs enable artists to generate an unprecedented range of visual outputs, from lifelike landscapes to surrealistic portraits, thereby expanding the canvas of human creativity.
- Redefinition of Authorship: Using GANs introduces complex questions about the origin of artistic ideas as the algorithm collaborates in the creative process, challenging traditional notions of authorship.
- Inspiration for Human Artists: By providing a new toolkit for exploration, GANs serve as creators and muses, inspiring human artists to reinterpret their visions and methodologies in light of AI’s capabilities.
These implications underscore the transformative role of GAN technology, as it continuously reshapes the terrain of artistic innovation and expression.
Ethical Considerations
As we delve into the ethical landscape surrounding Generative Adversarial Networks (GANs), we must consider how their capabilities might be misused, necessitating a framework for ethical application to prevent potential misuse. The emergence of GANs has brought forth a plethora of creative possibilities in the realm of AI art, yet it has equally spawned avenues for the creation of misleading or harmful content. The potential weaponization of GAN technology, specifically through the production of deepfakes, underscores the urgent need for ethical guidelines that govern their use.
Integrating responsible practices in deploying GANs is paramount to mitigate risks associated with their output. Ethical considerations must be at the forefront to ensure the technology is not exploited to deceive, harm, or perpetrate fraud. Furthermore, the advent of GANshare One, which contemplates the commercialization of shares in a trained GAN, complicates the ethical and legal landscape. This development raises questions about ownership, intellectual property rights, and the implications of commodifying access to generative models.
Thus, engaging in a technical, analytical, and precise discourse on the ethical considerations of GAN technology is critical, focusing on establishing a balance between innovation and preventing its potentially detrimental effects.
The Future of AI Art
As we consider the trajectory of AI art, integrating GAN technology raises critical ethical implications, particularly regarding the authenticity and ownership of AI-generated works.
Collaborative efforts between human artists and AI systems are poised to redefine the creative process, potentially leading to a new artistic expression and innovation paradigm.
Moreover, the disruptive potential of AI within the art market cannot be understated, as it introduces novel methods for creation, distribution, and monetization that may challenge traditional economic models.
Ethical Implications of AI-Generated
Delving into the ethical landscape of AI-generated art, one must confront complex issues of authorship, ownership, and the very nature of creativity. The ethical implications of AI-generated artwork are multifaceted and require nuanced consideration:
- Authorship and Ownership: Determining the rightful claimant to creative works when algorithms are involved blurs traditional boundaries of intellectual property rights.
- Consent and Privacy: Utilizing data, especially personal imagery, in generating art without explicit consent poses significant privacy concerns that must be addressed through stringent ethical standards.
- Commercialization and Accessibility: The surge of AI art in markets, especially as NFTs, prompts critical examination of the inclusivity of these platforms and the potential for creating new economic divides in the art community.
These points underscore the necessity for developing robust frameworks to navigate the ethical complexities introduced by AI in the realm of art.
Human-AI Creative Collaboration
While the ethical implications of AI art production raise essential questions, the collaborative relationship between human artists and AI, particularly GAN technology, heralds a new era in creative expression and innovation. Human-AI creative collaboration leverages the strengths of both entities; artists provide creative direction and emotional depth, while GANs contribute with their vast computational capabilities and data processing. This synergy is not only propelling the boundaries of art but is also steering innovation across various sectors.
Advantages | Challenges |
---|---|
Novel Perspectives | Ethical Considerations |
Enhanced Creativity | Misuse Potential |
Cross-Industry Impact | Content Authenticity |
The intersection of human ingenuity and AI’s algorithmic precision in GANs forms a cornerstone for groundbreaking advancements, albeit necessitating a vigilant approach to the ethical contours of this partnership.
Art Market Disruption Potential
The burgeoning presence of AI-generated art, mainly through the advent of NFTs, portends a significant transformation in the dynamics of the traditional art market. AI’s art market disruption potential is multifaceted:
- Democratization of Art Creation: AI empowers individuals to create and distribute art without traditional gatekeeping, altering market entry dynamics.
- Redefinition of Value and Authenticity: Digital scarcity, enabled by NFTs, challenges conventional notions of artistic value, authenticity, and ownership.
- New Revenue Models: Artists can leverage AI to explore novel monetization strategies, including algorithmically generated pieces and dynamic NFTs.
This paradigm shift, driven by technological innovation, compels a reevaluation of established art market structures and opens a discourse on the future trajectory of art collection and curation.
Case Studies: GANs in Action
Exploring real-world applications, Generative Adversarial Networks (GANs) have facilitated a variety of innovative projects across multiple domains, exemplified by the following case studies. Since their inception in 2014, GANs’ dual-structured framework, comprising a Generator and a Discriminator, has been pivotal in synthesizing realistic images through a strategic generative process.
One prominent case study: GANs in action is their transformative role in digital art creation. Groundbreaking projects have emerged, harnessing GANs’ capability to generate original artworks and challenging traditional notions of creativity and authorship. These algorithm-driven pieces have garnered attention for their complexity and aesthetic appeal, sparking discussions on the intersection of art and AI technology.
GANs have revolutionized content generation in the gaming sector by creating detailed textures and environments, enhancing the immersive experience without extensive manual labor. The healthcare industry has also benefited from GANs, which assist in medical imaging and data augmentation, contributing to advanced diagnostic tools and treatment planning.
Despite these advances, GANs present challenges like mode collapse and significant data requirements. Moreover, the potential for misuse underscores the importance of responsible deployment, ensuring ethical standards are upheld in this rapidly evolving field.
FAQs
Can artists control the style or theme of AI art generated by GANs?
Artists can influence the style or theme of AI art generated by GANs through the choice of training data and the tuning of model parameters. Controlling these aspects allows artists to guide the GAN towards producing art in specific styles or themes.
Are there pre-trained GAN models available for AI artists to use?
Yes, pre-trained GAN models are available for various applications, including AI art generation. These models can be used as starting points, allowing artists to build upon them or fine-tune them for their specific artistic goals.
What challenges do artists face when using GANs for AI art creation?
Challenges may include finding the right balance in training to avoid overfitting or underfitting, addressing potential biases in the training data, and experimenting to achieve the desired level of creativity while maintaining control over the output.
Can GANs be used for collaborative AI art projects?
Yes, GANs can be used for collaborative AI art projects. Artists can share models, generate elements using GANs, and combine them in collaborative efforts to create unique and innovative artworks.
How can artists ensure ethical considerations when using GANs in AI art?
Artists can ensure ethical considerations by being transparent about using GANs, avoiding using biased or controversial training data, and respecting copyright and attribution principles. Being aware of the potential impact on the art community is also essential.