Unveiling the Magic: AI and Neural Artwork
In recent years, the intersection of artificial intelligence and art has given rise to a remarkable phenomenon: the creation of neural artwork that captivates the human eye with its complexity and beauty. At the core of this innovation is a sophisticated orchestration of algorithms and computational processes that mimic and sometimes extend the nuances of human creativity.
Neural networks, trained on vast datasets of artistic works, employ techniques such as style transfer and generative adversarial networks (GANs) to produce unique visuals reminiscent of the human touch in artistry.
The driving force behind this technology raises profound questions about the nature of creativity and the role of machines in artistic expression. These inquiries challenge our traditional understanding of art and beckon us to explore how AI continues redefining the boundaries between the artistically organic and the digitally engineered.
As we consider the intricate dance between data and algorithm that enables AI to generate such compelling artwork, one cannot help but wonder where the line between programmed code and genuine artistry begins to blur.
Key Takeaways
- Neural networks and AI algorithms are crucial in generating stunning neural artwork.
- Data sets, training, and algorithms are integral to the generative process of AI art.
- AI art challenges traditional notions of creativity and pushes the boundaries of artistic expression.
- Ethical implications arise regarding copyright, ownership, cultural appropriation, and biases in AI-generated artwork.
Understanding Neural Networks
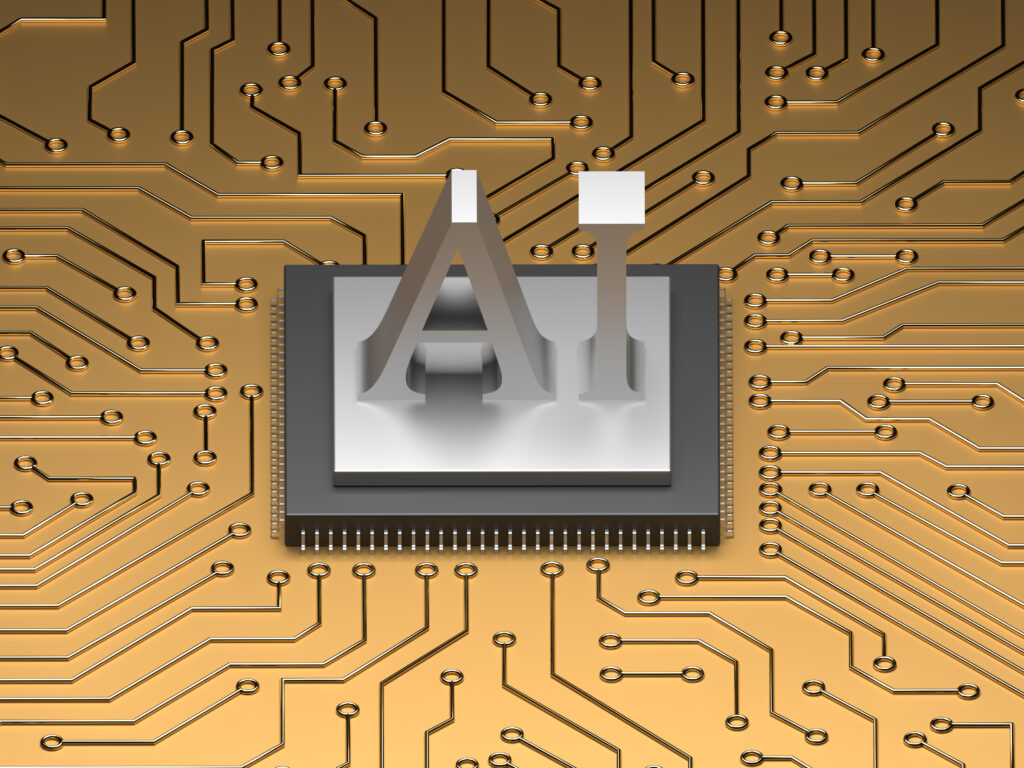
To fully appreciate the intricacies of AI-generated artwork, it is crucial to delve into the mechanisms of neural networks, which are complex computational systems inspired by the human brain’s architecture. These multi-layered networks form the cornerstone of AI Art Generation, harnessing the power of machine learning algorithms to emulate creative processes.
Through deep learning, a subset of machine learning, neural networks generate new images, analyze and interpret extensive training data, and assimilate patterns, styles, and textures.
Generative AI, particularly generative adversarial networks (GANs), is pivotal in advancing AI creativity. A GAN consists of two competing neural networks: a generator, which creates images, and a discriminator, which evaluates their authenticity. The algorithm is trained iteratively; with each cycle, the generator strives to produce increasingly convincing artwork, while the discriminator enhances its ability to detect nuances separating generated art from the training data.
Meanwhile, style transfer algorithms employ convolutional neural networks to extract and recombine artistic elements from different sources. They meticulously distinguish and manipulate the content and style of images, offering a synthesis that contributes to the diversity and sophistication of AI art generation.
The efficacy of these neural networks to generate new and compelling art significantly depends on the variety and richness of the training data provided.
Data Sets and Training
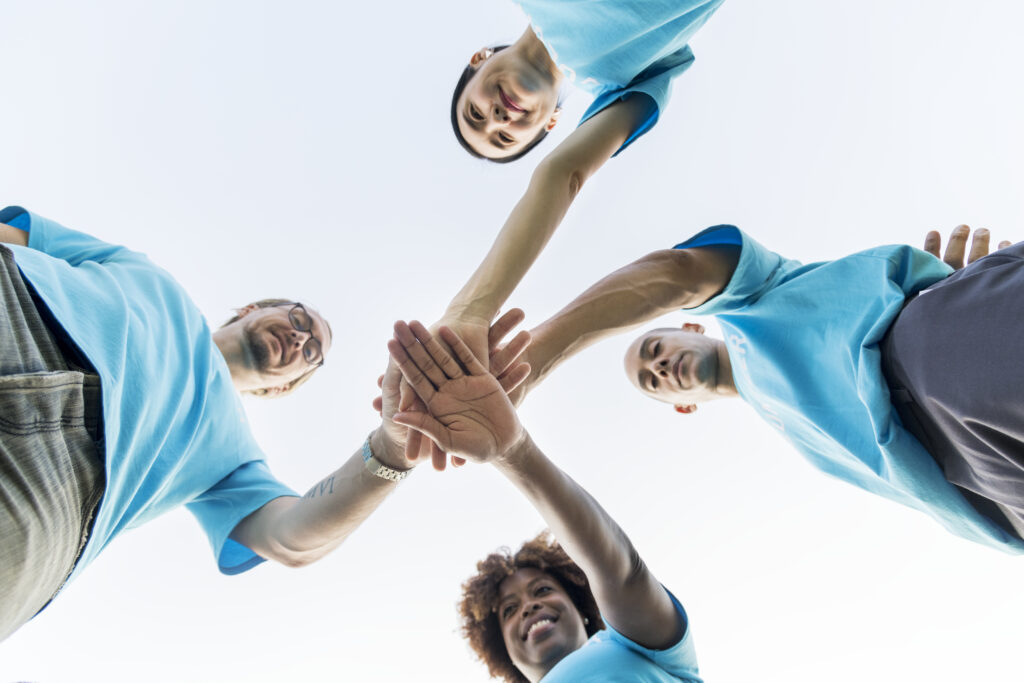
Data sets, encompassing vast arrays of human-crafted images, are integral to training AI algorithms, shaping the caliber and variety of the resultant neural artwork. AI art generators rely on these data sets to inform their neural networks on generating new images. The data used to train these models must be extensive and varied to ensure the creation of unique and compelling original works of art.
- Training Process:
- Large datasets of images are fed into the generative model, which learns patterns and features.
- Neural networks apply learned concepts to create new data, synthesizing previously unseen images.
- The iteration cycle includes adjusting parameters to refine the style image and output quality.
- Role of Prompt Engineering:
- Prompts are used to train AI art generators, guiding the neural network in generating specific themes or styles.
- Skilled prompters utilize emotion and color palettes to influence the generative process.
- Continuous prompt variation leads to a richer, more diverse set of generated artworks.
- Ethical and Quality Considerations:
- Training data selection involves copyright and ownership scrutiny to ensure ethical use.
- The quality and diversity of data sets directly impact the neural network’s ability to produce aesthetically pleasing art.
- Ongoing analysis of generated images is crucial for maintaining the integrity and evolution of the AI art generator’s capabilities.
Algorithms Behind the Art
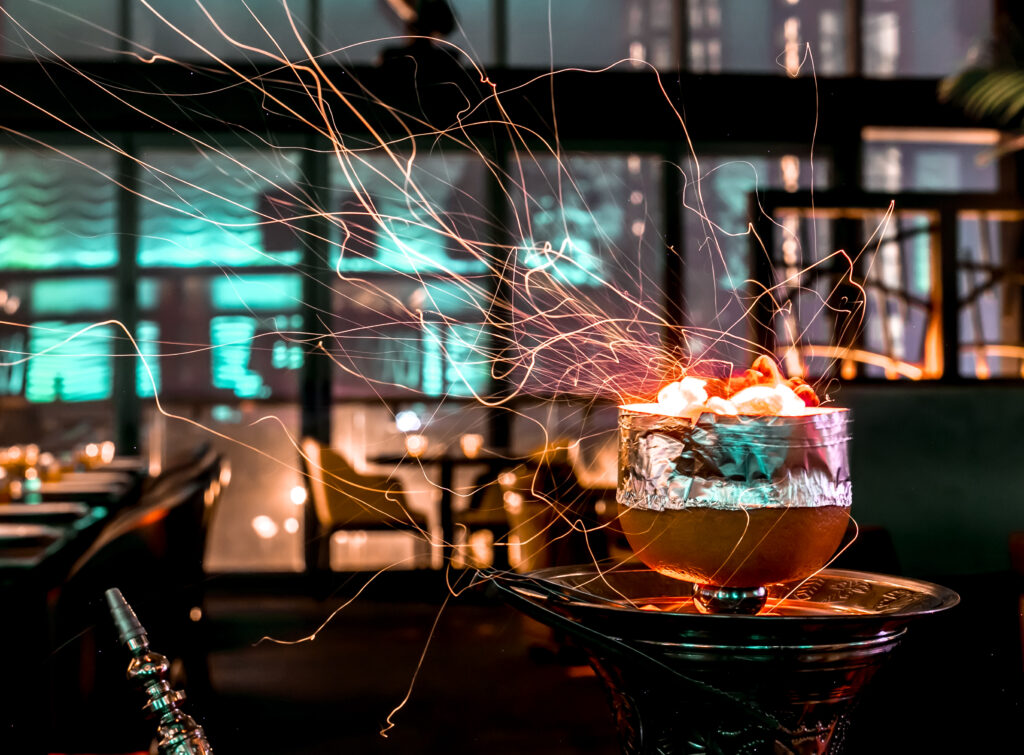
Having established the foundational role of data sets in training AI to produce neural artwork, we now focus on the sophisticated algorithms that drive the creation of these digital masterpieces. At the heart of these algorithms are neural networks inspired by the human brain’s interconnected structure, which enable artificial intelligence (AI) to generate art with unprecedented complexity and creativity.
Generative Adversarial Networks (GANs) are particularly influential in AI image generators. These consist of two parts: the generator, which creates images, and the discriminator, which evaluates them. GANs refine the generated artwork through a dynamic competition process, leading to increasingly realistic results.
Diffusion models represent another frontier in the use of AI to create art. These models iteratively modify a random signal until it forms a coherent image that resembles the training data, effectively ‘diffusing’ the randomness into structured, visually appealing patterns.
The following table illustrates the key components and characteristics of these algorithms:
Component | Function | Outcome |
---|---|---|
Neural Networks | Process and interpret vast data sets | Foundation for creating art with AI |
GANs Generator | Initiates the creation of art | Produces initial generated artwork |
GANs Discriminator | Assesses and guides refinement | Enhances the quality of AI-generated art |
In essence, the interplay of these algorithms embodies the generative process of using AI to produce art that resonates with both the chaos and the order found in human creativity.
Creativity and Machine Learning
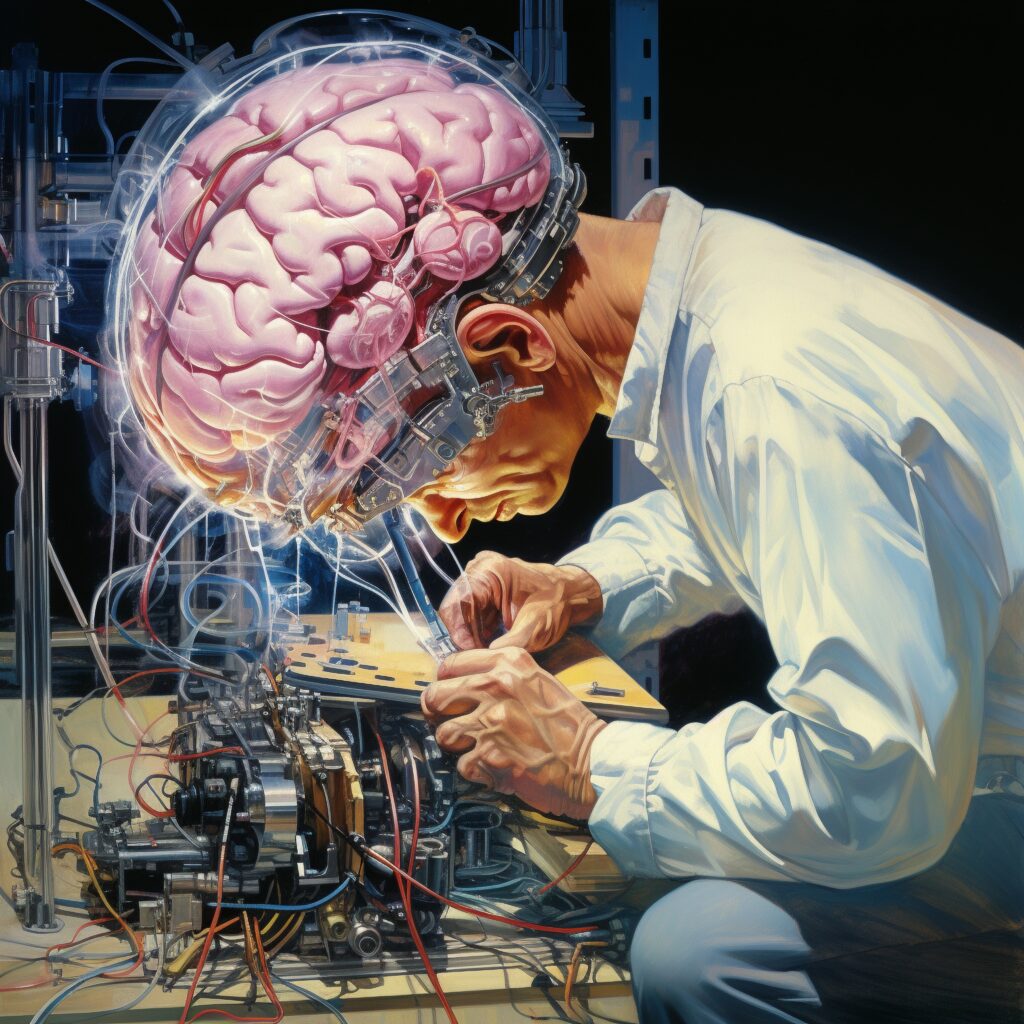
The intersection of machine learning and artistic creation has given rise to a novel paradigm of algorithmic creativity. AI systems, trained on vast datasets of creative works, can generate images that transcend traditional human concepts of art. These neural networks leverage complex algorithms to synthesize elements from diverse artistic styles. The result is a unique visual narrative that challenges our understanding of creativity’s boundaries.
The capacity of AI to generate such artwork pushes the envelope of artistic expression. It suggests that machine learning tools may augment and redefine the scope of human creativity.
Algorithmic Creativity Unleashed
In digital creativity, artificial intelligence systems can now produce artworks that push the boundaries of traditional human creativity, leveraging machine learning to generate visually captivating and unique images.
AI algorithms can be categorized into different types. Generative algorithms utilize deep learning to synthesize novel art, while neural networks analyze vast datasets, learning aesthetic patterns for art generation. Deep learning empowers AI with the ability to reinterpret and transform visuals.
The creative process fueled by artificial intelligence marks a significant shift in artistic expression. The technology facilitates an innovation-rich environment where artistic output is prolific and infused with complexity and nuances that may elude human artists.
The restructuring of images by AI introduces unprecedented perspectives and details, enhancing the spectrum of visual communication. AI-generated images populate realms previously void of visual representation, broadening the scope of where and how art is experienced.
Training on Artistic Data
Training AI models on artistic data harness the creative synergy between algorithmic precision and human aesthetic sensibilities, enabling the generation of novel and intricate artworks. The meticulous process involves AI generators that use neural networks to generate new art styles by training on vast datasets of artistic imagery. This training allows AI to learn and replicate complex patterns and aesthetics intrinsic to human-created art and, subsequently, to create new, unprecedented art pieces.
Key ElementRole in Art Generation | |
---|---|
Generative Models | Core engines used to generate art by learning data distributions |
Artistic Data | Core engines are used to generate art by learning data distributions |
Algorithmic Process | Ensures precision and diversity in generated artwork |
Ethical Considerations | Guides the responsible use of art data and outputs |
The output’s originality and aesthetic appeal are direct results of the quality and variety of the data used in training, affirming the hypothesis: in art generation, data is as critical as the algorithm itself.
Beyond Human Artistic Limits
Artificial intelligence transcends traditional artistic boundaries by leveraging machine learning to create visual masterpieces unbound by human imagination constraints. The use of AI in art represents a paradigm shift; AI tools are not merely extensions of human creativity but are entities capable of generating new forms and aesthetics that humans may never conceive.
- Impact on the Art World:
- AI-generated art influences new genres in games, movies, and metaverses.
- Raises questions about the visibility and relevance of human-created art.
- Creativity and Machine Learning:
- AI systems produce new art by learning from vast datasets.
- The generative type of AI can surprise even the artist who wants to explore beyond their style.
- Beyond Human Artistic Limits:
- AI can create an immense volume of diverse artwork, challenging our traditional notions of creativity.
Ethical Implications
The proliferation of AI-generated neural artwork precipitates complex ethical debates surrounding artistic copyright concerns, particularly in determining the locus of originality and ownership between human creators and algorithmic processes.
Concurrently, the emergence of AI systems capable of producing art instigates discussions on AI consciousness and its implications for attributing artistic merit or legal rights.
Furthermore, the potential for such systems to engage in cultural appropriation, inadvertently reflecting and amplifying biases from their training datasets, poses significant ethical challenges that demand careful consideration and potential regulatory measures.
Artistic Copyright Concerns
Amid the rise of AI-generated imagery, contentious legal and ethical questions surface regarding the rightful ownership of artistic copyright.
- Copyright Ownership:
- The extent to which AI-generated works, using text prompts to emulate an image’s style, can be owned as intellectual property remains ambiguous.
- Human involvement in directing AI impacts claims over the final image, complicating traditional copyright norms.
- Ethical considerations arise when AI recreates visual elements reminiscent of authentic images copyrighted by human artists.
The analytical approach to these issues demands a technical understanding of how AI operates: the algorithms processing inputs to create outputs distinct from yet inspired by existing artworks.
The precise delineation of ownership rights over these AI-created works is pivotal, as it influences the integrity and evolution of legal and artistic landscapes.
AI Consciousness Debate
While navigating the complexities of copyright ownership in AI-generated art, a parallel discourse emerges regarding the ethical implications of potential AI consciousness. The consciousness debate intensifies as AI begins to make art that rivals the content of the content produced by human brains, challenging the unique capabilities traditionally attributed to the human artist. Can generative art be considered true art, or will it always lack the subjective experience and intentionality that define human creativity?
Ethical Concern | AI Consciousness Aspect |
---|---|
Autonomy | Self-directed creativity |
Accountability | Attribution of artwork |
Rights | Ownership and copyright |
Moral Treatment | Empathy towards AI |
Human Exceptionalism | AI’s role in art |
The implications of AI potentially replacing human artists in creating art raise profound questions about the nature of consciousness and the value we ascribe to human-centric creativity.
Cultural Appropriation Risks
The potential for cultural appropriation in AI-generated artwork introduces a complex layer of ethical considerations that necessitate a nuanced understanding of cultural sensitivity and intellectual property rights. The deployment of generative AI in creating new artwork must be conscientious:
- Art must:
- Respect the cultural origins of existing art
- Recognize the creativity and labor made by humans that informs AI datasets
- Ensure that the images created do not perpetuate harmful stereotypes or exploit cultural symbols
- Cultural appropriation risks involve:
- The commodification of cultural elements without proper attribution or consent
- The potential erasure of cultural narratives when repurposed in generative artwork
- The undermining of cultural heritage through the unacknowledged use of sacred or significant motifs
- Generative AI systems designed to generate something new should:
- Integrate ethical guidelines that prevent cultural insensitivity
- Foster collaboration with cultural stakeholders
- Promote transparency in the type of productive processes and sources used
The Future of AI Artwork
Exploring the trajectory of AI-generated artwork, industry experts predict a significant impact on traditional artistic production and the broader creative economy. As new algorithms, such as Stable Diffusion, are developed, the potential for AI to produce images that rival and even surpass human creativity continues to grow. These algorithms and methods follow a series of steps that start with a content image and progressively refine it, leading to more detailed photos.
AI systems are improving at creating more realistic images and exhibiting a level of detail that could be described as hyper-realistic. The images generated by these systems are becoming increasingly indistinguishable from those made by human hands, which suggests that the future of AI artwork will likely include a blend of human and machine creativity.
Aspect of AI Artwork | Future Potential |
---|---|
New Algorithms | More sophisticated AI art with enhanced realism and complexity. |
Production Scale | A synergistic relationship between human artists and AI, leads to novel artistic expressions. |
Creative Collaboration | A synergistic relationship between human artists and AI leads to novel artistic expressions. |
As AI evolves, the art industry may witness a transformative shift where the boundary between human-made and machine-generated artwork becomes increasingly blurred.
FAQs
What is the underlying technology that drives AI to generate neural artwork?
The underlying technology often involves neural networks, particularly Generative Adversarial Networks (GANs), which consist of a generator and a discriminator. GANs learn from data to create new and visually appealing images.
How do Generative Adversarial Networks (GANs) contribute to generating stunning neural artwork?
GANs contribute by employing a generative process where the generator creates images, and the discriminator evaluates their realism. The adversarial training between these components results in the generation of visually stunning and diverse artworks.
What drives the creativity in AI-generated neural artwork?
The creativity in AI-generated neural artwork is driven by the training process, where the neural network learns patterns, styles, and features from a diverse set of training data. The network then uses this knowledge to generate new and imaginative artwork.
Can AI be programmed to mimic specific artistic styles or genres?
Yes, AI can be programmed to mimic specific artistic styles or genres by training the neural network on datasets containing artworks with those characteristics. This allows the AI to learn and reproduce the unique features of the selected styles.
What role does the training dataset play in the quality of AI-generated neural artwork?
The training dataset is crucial in determining the quality and style of the AI-generated neural artwork. A diverse and representative dataset helps the neural network learn a broader range of features and create more versatile and stunning artwork.